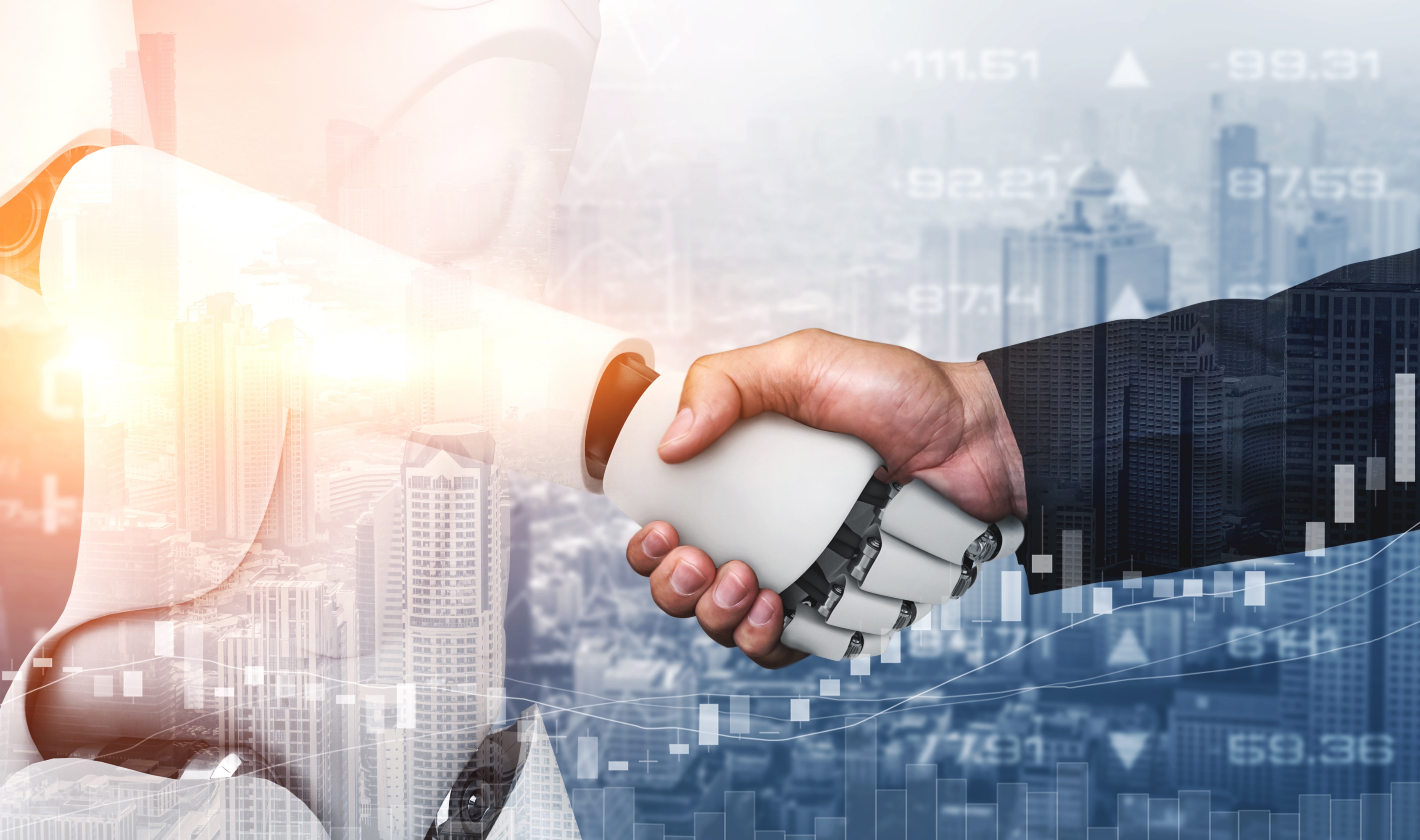
Without a doubt, artificial intelligence has become a driving force of the digital revolution. Businesses worldwide are adopting AI as part of their digital transformation journey, “not because they can but because they must to be agile, resilient, innovative, and able to scale”. However, it was clearly not an easy road.
According to an Internationa Data Corporation (IDC) global survey in 2019, most organizations reported failures among their AI projects, with a quarter of them reporting up to a 50 percent failure rate. While every business has its own methodology and approach to AI adoption, these are some of the most recurring issues that have been observed by adopters across verticals:
#1 Lack of a data strategy
Data is the fundamental building block for any AI initiative. A data strategy, according to IBM, entails how a business collects, stores, documents, manages and makes data accessible to the rest of the company. Unfortunately, a Forbes survey of 700 global C-suite executives shows that only 12 percent of them have built, and are executing, a company-wide data strategy. Analysts at Gartner estimate that more than 80 percent of enterprise data is unstructured, ranging from text files, emails, social media messages, to employee complaints. This makes it extremely challenging for businesses to centralize data from different systems of records and silos, increase visibility and accessibility of data across departments, and address data management aspects such as data quality, data security, among many others.
So, it helps to think data first before thinking AI. AI solutions built on a cohesive, well-structured data strategy will not only give businesses the power to “see” and understand customers, but also be able to predict trends in the market, thereby continually updating strategies as they grow.
Read more: A Few Thoughts on AIs and Its Impacts on Our Future
#2 No linkage to business outcomes
Another reason why AI projects underperform for certain businesses is that they tend to be isolated from business activities. A survey from MIT Sloan Management Review and Boston Consulting Group released in October 2019 found that it is a significant gap that seperates “winners” and “losers” when it comes to AI adoption.
To a large extent, companies failing to generate tangible results from artificial intelligence view AI as merely “a technology thing” without considering how the organization will get and use the AI results. They seem to focus more on the production of AI, e.g. setting up tools, systems, and processes to enable AI deployment, according to the survey findings. In stark contrast, the most successful AI adopters define business pain points and challenges in advance. They align business owners, process owners, and AI experts to ensure that they adopt AI solutions effectively.
A well-defined AI strategy should glue technology and business together. Top management should kickstart the process by defining what business problems that need to be solved, why they want to incorporate AI into their workflows, what they hope to accomplish and what success will look like.
#3 Lack of talents
Between 2012 and 2017, the number of data scientist jobs on LinkedIn increased by more than 650 percent. However, the supply of competent practitioners seemed not to measure up, and it hurt businesses that wanted to adopt AI.
The lack of AI talents is urging businesses to retrain and upskill their existing employees. A Mindtree study survey of 650 global IT leaders in 2019 found that the most sought-after skills for AI adoption include design thinking (58 percent), data engineering (58 percent), and data science (54 percent ). While 47 percent of businesses in the survey said they are in the process of retraining current staff members, more than half still need to reskill.
More reading on virtual workforce: Why RPA Shall Stop Being Optional for HR Departments
A few last thoughts
AI can spur serious growth—but only if it is done right. Successful AI adoption requires a much broader perspective that incorporates not just the technology itself, but also a business-oriented mindset, a comprehensive data structure, investment in talents, and so on. As Terry Hickey, Chief Analytics Officer at CIBC said, AI projects should be viewed as “snacks, not meals”. The trick is to “make them big enough to get people excited and to show the potential of AI, but small enough that they can be implemented quickly”.
References:
1. IDC survey finds artificial intelligence adoption being driven by improved customer experience, greater employee efficiency, and accelerated innovation [Link]
2. Five steps to building a data strategy for AI [Link]
3. Behind every AI strategy is a data strategy [Link]
4. Annual MIT Sloan Management Review-Boston Consulting Group study finds few organizations realizing value from AI [Link]
5. Don't make these mistakes when investing in AI [Link]
6. The real reason businesses are failing at AI [Link]