Whitepaper Overview
We propose in this paper a deep learning method for human density estimation, targeted to sparse density scenarios. We divide the image into a grid of cells and realize that a person does not span in one cell but several cells. To estimate the human density that appeared in one cell, the information of surrounding cells plays a crucial role, so-called spatial contextual information. The key insight of our approach is the employment of Convolutional Neural Networks (CNN) to extract image features and Long Short-Term Memory (LSTM) to exploit the spatial contextual information. The shared-weight mechanism of CNN models generalizes the learned features of humanity in all corners of the images. The feedback mechanism of LSTM captures spatial contextual information of neighboring cells in a sub-grid of 3x3 cells to estimate the human density of the central cell. We demonstrate the validity of the proposed method on the Mall dataset with a competitive performance in comparison with state-of-the-art methods, validated on the same dataset.
Preview
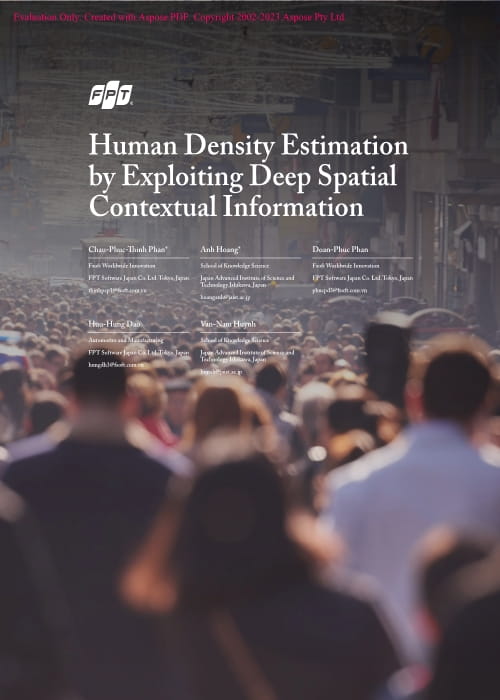
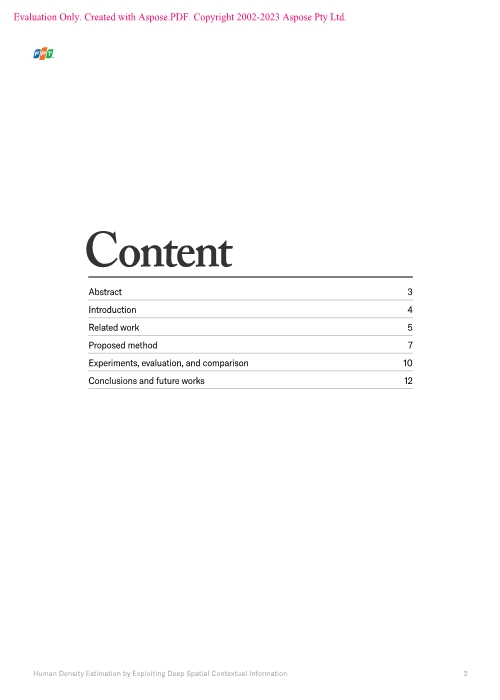
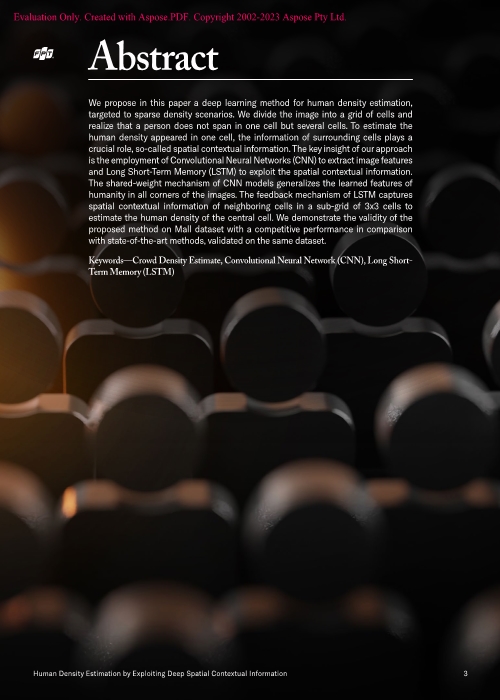
Download Full Version of the White Paper
Free Download