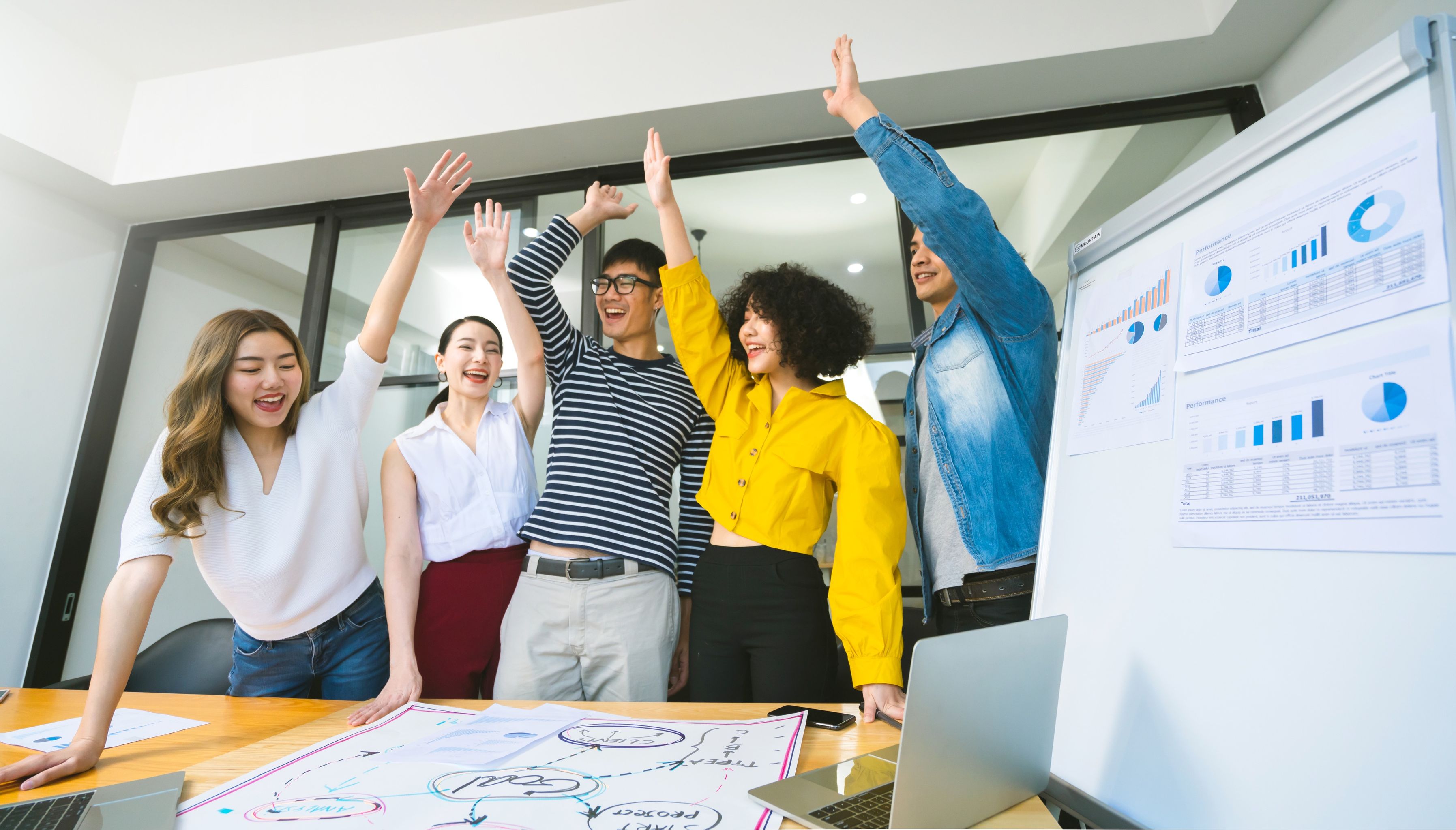
Organizations are turning to predictive analytics to help uncover new opportunities and address challenging issues.
A key pillar of data analytics
Predictive analytics is a subset of advanced analytics that forecasts future developments using historical data, statistical modeling, data mining, artificial intelligence, and machine learning. While the technology has been around for decades, organizations have not turned to it until recently. With the boom of data mining, data analytics, and intelligent software, companies found advantages in uncovering patterns and relationships based on data. These patterns are then used to create tactical models that lower risk, streamline processes, and boost productivity.
As decision-making by both humans and AI is becoming increasingly complex and demanding, predictive analytics have become more powerful than before. This prompts enterprises to be more reliant on data-powered, deep-learning approaches. According to a report conducted by Future Market Insights Inc, the predictive analytics market is expected to reach a valuation of US$55.5 billion by the end of 2032 [1].
Transforming data into future insights
Mainly utilized to increase a company's bottom line and competitive advantage, predictive analytics allows enterprises to find patterns in past data to build models that project future outcomes by applying algorithms to large data sets. These data sets might consist of unstructured and structured data, such as transactional databases, texts, images, videos, audio, sensor records, log files, and more. While all predictive models can be seen as replications of real-world scenarios, the type of models vary widely depending on the company's objective. Some common predictive models include:
- Regression models are used to evaluate the strength of a relationship between variables and how they impact future outcomes. For instance, organizations can determine how the quality of their products may affect the end customers' shopping decisions.
- Classification models are favored by financial institutions for their ability to identify fraudulent transactions by analyzing millions of past transactions to segregate risky customers from risk-free ones.
- Clustering models thrive on data matrices that cluster similar data with the same features to identify hidden insights. Such models help businesses to create more personalized, target-focus strategies.
- Time-series models are commonly used to predict sales, stock prices, or weather patterns. These models are unique from others because they take the time-based nature of data into account. It can show how the data regulates over the course of data points as well as the end outputs.
In short, predictive analytics help businesses stay informed, detect fraud, thrive on future opportunities, and serve customers better. It brings forth actionable insights for enterprises to get ahead of the competition. In the long run, analytics initiatives can lower ongoing expenses and reduce wasted capital through workflow optimization and more throughout campaign planning.
The potential drawbacks, however, are the time and data-gathering efforts it takes for predictive analytics to effectively produce meaningful results. If the predictive models are not fed with reliable, high-quality data, the outturns will bounce back inaccurately. Having clean data is particularly acute because bad information can cost businesses up to US$15 million a year in losses [2]. For analytics to become more reliable as time goes on, it is best to obtain data from reputable, trusted sources and verify this information against other sources.
The possibilities are theoretically endless
There are numerous possible applications of predictive technology out there, but the fundamental values of analytical tools are best captured through the major use cases that exist in industries today.
- BFSI - fraud detection and prevention: The banking sector has long used predictive analytics to identify and eliminate fraud, assess credit risk, and maximize cross-sell and up-sell opportunities. After all, the power lies in its ability to recognize patterns. This means it can detect any unusual patterns of behaviour should they occur. Simply put, if a banking customer based in the UK seems to be making a transaction in several other continents within a short period of time, the bank will be flagged for security intervention.
- Marketing - behavioural targeting: Consumer data is widely available in marketing and is used to develop content, adverts, and more effective strategies for reaching potential customers wherever they are. Predictive analytics can be employed to anticipate seasonal sales trends and organize promotions accordingly. It can help businesses build a deeper understanding of their customers by providing insights into consumers' preferences and forecasting a lead's propensity to progress from awareness to purchase.
- Manufacturing - predictive maintenance: The goal of predictive maintenance solutions is to predict when equipment failure would happen and how to prevent such breakdowns by pre-scheduling corrective maintenance. Such prediction reports are shared with the respective stakeholders so proactive actions and decisions can occur. Generally, this makes the overall operations efficient and rewarding. Having a functional predictive maintenance program can help manufacturers lessen 70-75% chances of machinery breakdowns by reducing maintenance costs by up to 30% and ten times increase in ROI [3].
According to a 2017 analysis published by Zion Market Research, predictive analytics has gained the backing of a broad spectrum of companies. The global market is expected to reach $10.95 billion by 2022, expanding at a compound annual growth rate (CAGR) of almost 21% between 2016 and 2022 [4]. Whether it is to drive financial decisions, formulate marketing strategies, or modify the course of action, building a foundation in analytical skills can serve the business well in the near and extended future.