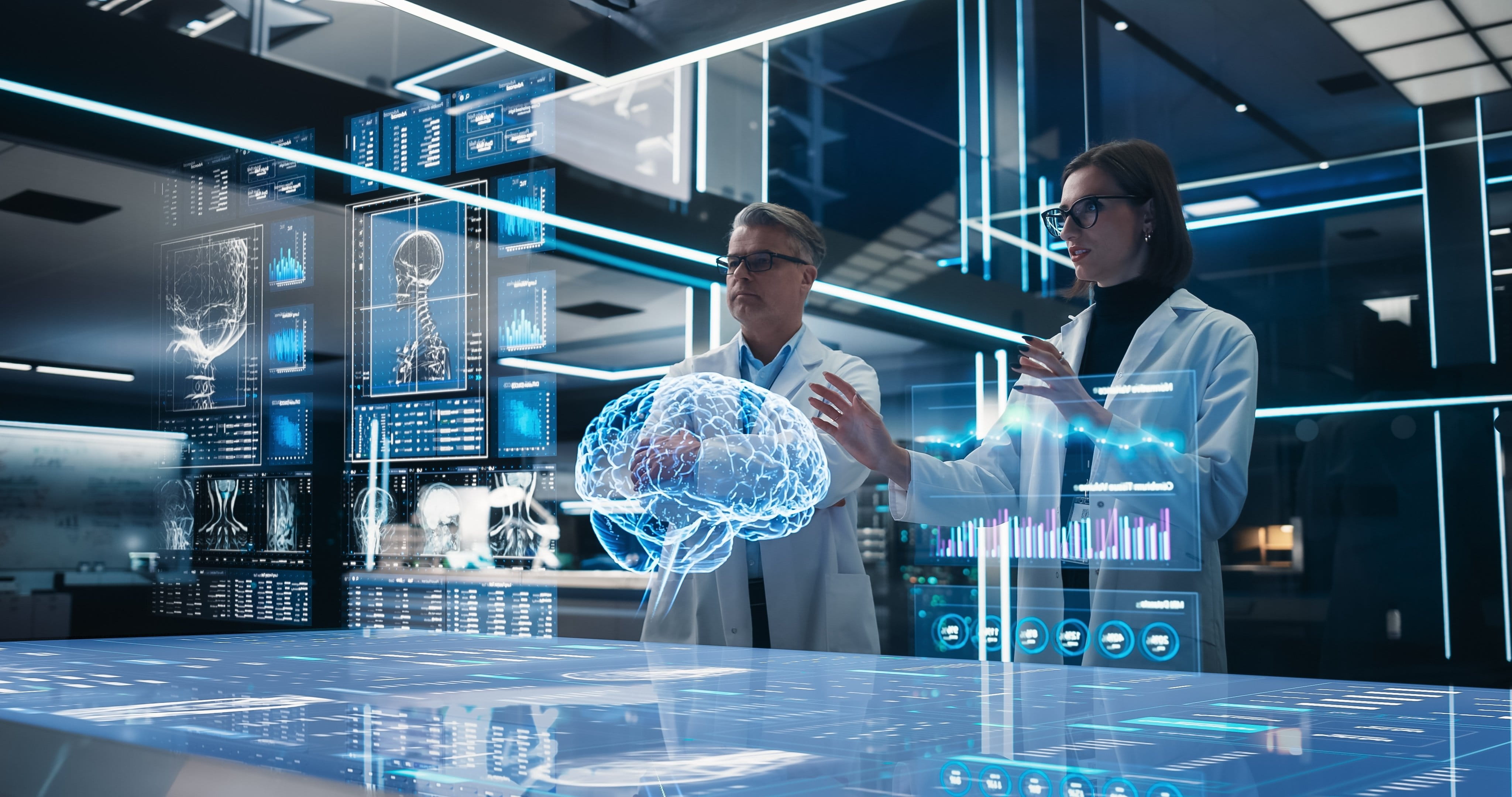
Deep learning, also known as deep structured learning, is a type of machine learning that uses a layered algorithmic architecture to analyze data. According to Grand View Research, the global AI in healthcare market size is expected to expand at a compound annual growth rate (CAGR) of 37.5% from 2023 to 2030 [1]. Specifically, deep learning is a subset of AI that focuses on the use of deep neural networks for solving complex tasks. For healthcare, deep learning stands as a promising force thanks to its capabilities:
- Drug discovery and precision medicine;
- Supporting clinical decisions; and
- Analyze imaging and diagnostics
1. Drug discovery and precision medicine
Precision medicine and drug discovery require processing vast amounts of genomic, clinical, and population-level data. Their aim is to uncover previously unknown connections between genes, drugs, and physical environments. These unexplored datasets in drug discovery are intricate due to their sheer volume, diversity, and often fragmented nature. They may encompass various data types, such as genomics, proteomics, chemical structures, and clinical records, which are challenging to integrate and analyze comprehensively. Given the valuable insights and potential, deep learning is a valuable approach for researchers and pharmaceutical stakeholders to accelerate drug discovery.
Traditionally, drug discovery involves screening many chemical compounds to identify those with potential therapeutic properties. Now, deep learning models can analyze compounds' structural and chemical properties to predict their efficacy, thereby narrowing down the pool of candidates for further experimentation. Besides, deep learning models can predict a compound's biological activity based on its chemical structure. This helps researchers prioritize compounds more likely to have the desired pharmacological effects, reducing the need for extensive experimental testing. As the global AI in the drug discovery market is projected to attain a value of USD 7.1 billion by 2030 [2], healthcare professionals can harness the potential of deep learning to revolutionize drug development and streamline research processes.
Furthermore, deep learning models can predict the potential toxic effects of drugs early in the development process and prevent the advancement of compounds likely to have safety issues. Specifically, a study by University of Massachusetts researchers showcases the superiority of deep learning in identifying adverse drug events (ADEs) compared to traditional models. This tool sifts through unstructured electronic health record (EHR) data by combining deep learning with natural language processing (NLP). In this process, NLP helps extract valuable information from EHR and record the progress of clinical trials by analyzing reports. Specifically, it highlights correlations between medication type, frequency, and dosage, which are valuable for monitoring new therapies' safety and understanding real-world pharmaceutical prescription patterns. Despite varying levels of adoption, there is broad consensus on the future potential of this technology, with more than 80% of current AI users (and 70% of current non-users) stating that they expect AI to drive significant impact in drug discovery over the next five years [3].
2. Clinical decision support
Deep learning can be pivotal in clinical decision support and predictive analytics across various medical conditions. Deep learning can quickly notify healthcare providers about critical changes, such as sepsis and respiratory failure. For instance, MIT's Computer Science and Artificial Intelligence Laboratory (CSAIL) is at the forefront of this movement with its ICU Intervene project. This initiative alerts clinicians to patient deterioration in critical care units by harnessing deep learning. Unlike previous approaches emphasizing mortality prediction, ICU Intervene suggests actionable treatments. It accomplishes this using a singular model that predicts multiple outcomes, enhancing efficiency.
Deep learning also offers clinicians comprehensive explanations for its recommendations, building trust and enabling providers to make decisions, even if they choose to override the algorithm's suggestions confidently. DeepMind, based in the UK, is pioneering clinical decision support for eye diseases. The company is developing a commercial deep-learning Clinical Decision Support (CDS) tool capable of identifying over 50 eye ailments and providing corresponding treatment recommendations. Supported by a study in Nature, DeepMind and Moorfields Eye Hospital validated the tool's accuracy, demonstrating its equivalence to human clinicians. This innovation can potentially revolutionize access to care by significantly reducing diagnostic time.
Optical coherence tomography (OCT) scans are traditionally used for diagnosing eye conditions. However, these scans require expert explanation and time-consuming analyses, potentially leading to delays or severe consequences for patients. DeepMind's deep learning solution expedites the triage process almost instantly, ensuring patients receive timely care without compromising quality. DeepMind expressed enthusiasm about this milestone, emphasizing the synergy between clinicians and technologists in advancing healthcare.
3. Imaging analytics and diagnostics
Convolutional neural networks (CNNs), a type of deep learning, can analyze images such as MRI results or X-rays. These networks are optimized for image processing, which allows them to work efficiently and handle large images. At present, CNNs can compete with, and sometimes even surpass, human diagnosticians in accurately identifying important features.
In June 2018, a study in the Annals of Oncology demonstrated CNN's capabilities. Trained on dermatology images, CNN exhibited a 10% higher specificity in identifying melanoma than human clinicians. Even when clinicians had patient background data, such as age, sex, and lesion location, CNN outperformed dermatologists by nearly 7% [4]. The researchers from various German academic institutions concluded that a CNN algorithm could aid physicians in melanoma detection, irrespective of their experience.
The speed of deep learning tools is equally remarkable. Researchers at the Mount Sinai Icahn School of Medicine engineered a deep neural network capable of diagnosing neurological conditions (such as stroke) 150 times faster than human radiologists [5]. The tool processed and analyzed images, signaling problematic findings in just 1.2 seconds. Among these groundbreaking achievements is the astonishing result of deep learning models in diagnosing diabetic retinopathy with 97% accuracy [6].
Powering healthcare with deep learning
Deep learning has rapidly emerged as a game-changer in healthcare, revolutionizing data analysis with unprecedented speed and precision in drug development and clinical decision support. Nevertheless, healthcare institutions must navigate the intricacies of deep learning to unlock its full potential.