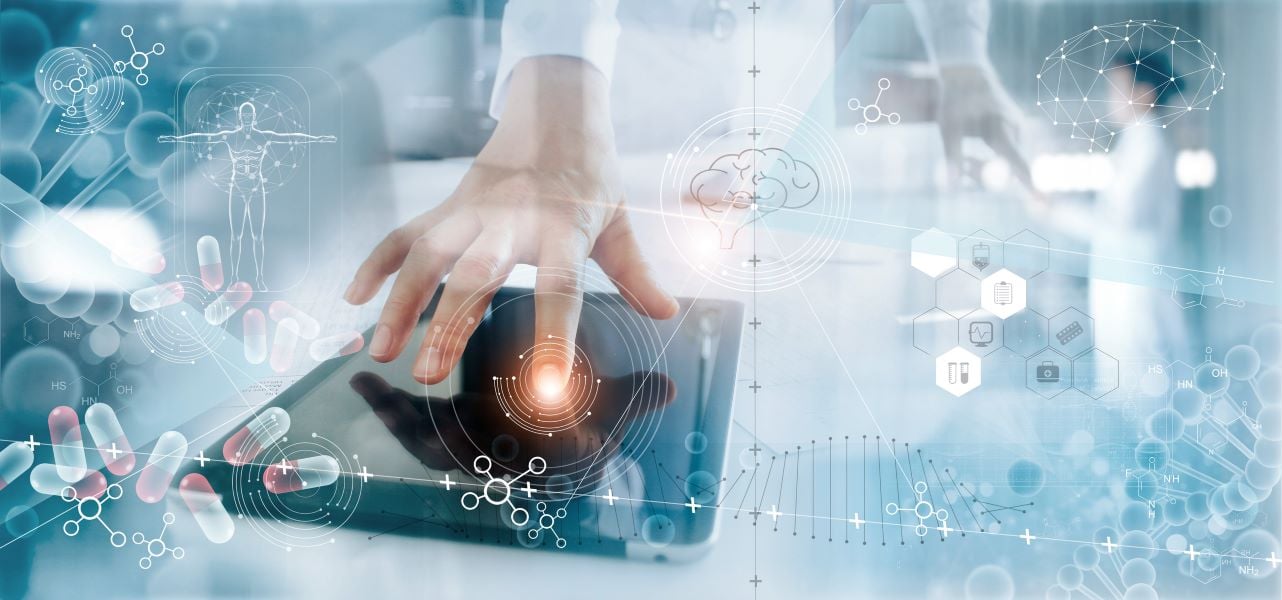
The adoption of generative AI - a prominent artificial intelligence system such as ChatGPT designed to create or generate new data that resembles a particular dataset it has been trained on - is witnessing remarkable growth in the healthcare industry. With estimates indicating that AI in the healthcare market will reach USD 102.7 billion by 2028 [1], generative AI is pivotal in driving healthcare innovations, despite potential bias concerns.
Streamlining Workflow with Accuracy
The healthcare industry faces ongoing struggles with administrative challenges that have far-reaching consequences. Notably, recent research highlighted that up to 50% of medical errors in primary care stem from administrative causes, such as staffing problems, documentation errors, equipment and technology failures, and lack of protocols and procedures [2]. Compounding these challenges is the global shortage of medical professionals, which poses a complex landscape for healthcare delivery. The World Health Organization (WHO) has projected a staggering shortfall of 10 million healthcare workers by 2030, predominantly affecting low- and lower-middle-income countries and further intensifying the difficulties in providing comprehensive care to all those in need [3].
Thus, generative AI presents promising opportunities for streamlining healthcare operations and helps tackle administrative challenges and talent shortages. In the training process, generative AI collects shift-handoff notes from different healthcare settings and eliminates irrelevant data. When a healthcare professional utilizes this tool, they will provide a prompt related to the patient's data. The AI model then predicts the continuation of the input based on its understanding of medical language and the patterns it has learned from the training data. By filling in the information gaps in the care coordination notes and shift-handoff notes, generative AI facilitates accurate communication among healthcare providers, enabling seamless care transitions and ultimately improving patient outcomes.
Furthermore, generative AI's ability to synthesize language holds significant potential in enhancing Electronic Health Records (EHR) workflows. While EHR systems grant access to vital patient information, they often rely on manual data entry, leaving room for human errors. Recognizing this issue, hospitals and physician groups are actively exploring the integration of generative AI across various EHR functions. This includes prepopulating visit summaries, suggesting documentation changes, and providing relevant research for decision support.
For instance, generative AI prepopulates visit summaries for healthcare by leveraging its language modeling capabilities and analyzing patient-specific data. When a patient's visit data is entered into the system, the AI model encodes the information to understand the context of the visit, including the patient's medical condition, treatment provided, and relevant diagnostic findings. Besides, generative AI can detect anomalies, perform data validation checks, and flag potential errors for review. Hence, AI-prepopulated data can reduce human errors by analyzing and correcting the data entry, such as typos, omissions, or incorrect values, thanks to its language modeling capabilities and contextual understanding. With manual data entry accounting for between 26% and 39% of healthcare workers' responsibilities [4], generative AI can alleviate this burden, freeing healthcare professionals from administrative tasks and empowering them to prioritize direct patient care.
Exploring New Horizons of Drug Development
The investment in AI solutions for drug testing has seen remarkable support from healthcare organizations. Gartner analysts highlight that venture capital firms have poured over USD 1.7 billion into generative AI solutions within the past three years, with AI-enabled drug discovery and AI software coding securing the most funding [5]. This substantial investment reflects the recognition of the pressing need to address the time-consuming and costly process involved in identifying potential drug candidates and assessing their efficacy and safety. Nevertheless, the drug development journey is characterized by a long development time of 10-15 years, with an average cost to bring a new drug to clinical use ranging from USD 1-2 billion [6].
Generative AI proves invaluable in expediting drug discovery processes by harnessing its ability to analyze vast molecular structure databases, predict properties, and identify potential drug candidates. Thanks to the large datasets of chemical structures and properties, this AI algorithm can generate novel molecules resembling existing drugs through learned patterns. Studies indicate that Computer-Aided Drug Design (CADD) significantly reduces drug development time, with conservative estimates suggesting that AI pipelines require less than one-third of the prevailing time [7]. Furthermore, AI simulations create new compounds faster, as the software platform efficiently tests millions of hypotheses. The departure from traditional trial-and-error methods offers a more accelerated approach to drug development. To alleviate the cost challenges, AI is also used to reduce the need for physical testing of candidate drug compounds by enabling high-fidelity molecular simulations that can be run entirely on computers without the high costs of traditional chemistry methods. According to research, AI can save the pharmaceutical industry up to 70% of drug discovery expenses [8]. By leveraging the power of machine learning and advanced algorithms of generative AI, researchers can explore infinite combinations of chemical molecules and uncover potential drug candidates that might have been missed using traditional methods.
Navigating the Paths to Unbiased AI
The emergence of generative AI has brought forth immense potential, particularly within the healthcare industry. However, the dangers of bias and discrimination in generative AI demand great caution from healthcare organizations. While the use of AI in medical image analysis, such as X-rays and MRI scans, has gained traction, these systems often contain implicit biases from their trainers, further exacerbating the biases. A study by Larrazabal et al. (2020) revealed that gender imbalances in training datasets for computer-aided diagnosis (CAD) systems led to markedly lower accuracy for underrepresented groups [9]. In simpler terms, when predominantly male X-rays were employed for training, the diagnostic accuracy for women's conditions dramatically declined.
Alarming concerns have also been raised by dermatology experts regarding the reliability of AI tools in evaluating skin lesions among individuals with skin of color, highlighting the broader implications of underrepresentation. Wen et al. (2021) shed light on significant limitations within open-access datasets for skin cancer images. Specifically, out of a total of 106,950 images analyzed, only a small fraction contained recorded information about skin type, with a significantly low representation of individuals with darker skin tones [10]. Furthermore, no images were available from individuals of African, African-Caribbean, or South Asian backgrounds. Although individuals with darker skin are generally less susceptible to skin cancer, the American Academy of Dermatology Association highlighted that "when skin cancer develops in people of color, it is often diagnosed at a more advanced stage, making it more difficult to treat [11]." Due to the lack of diversity in AI training, algorithms encounter significant challenges in identifying skin cancer in patients with darker skin tones, resulting in missed diagnoses or incorrect assessments.
To tackle these pressing challenges, business leaders need to establish diverse AI developer teams during the development phase. This diversity of perspectives can help mitigate bias in the process. Furthermore, regulatory actions by government and academic entities at an industry level are essential to ensure fair and unbiased AI development within the healthcare sector. Given the sensitive nature of medical data, concerted efforts must be made to ensure the availability of high-quality data that is both robust and impartial for training AI models. Furthermore, embracing a data-centric approach to AI development can significantly contribute to addressing biases in AI systems. This approach prioritizes data quality, diversity, and fairness during AI model training, ensuring the production of relevant and representative data while also addressing any inherent biases present in the data. By doing so, it fosters equitable healthcare outcomes for all, promoting fairness and inclusivity in healthcare AI applications.
Towards a Promising Future of Healthcare
While generative AI is poised as a potential solution to offer great benefits in streamlining workflow and accelerating the drug discovery process, it is crucial to note that this technology is still evolving. The output accuracy of generative AI depends on the quality of the datasets used to train them, including medical records, lab results, and imaging studies. Hence, the healthcare industry has to address these challenges to ensure the technology's ethical use, improve healthcare outcomes, and ultimately benefit patients.