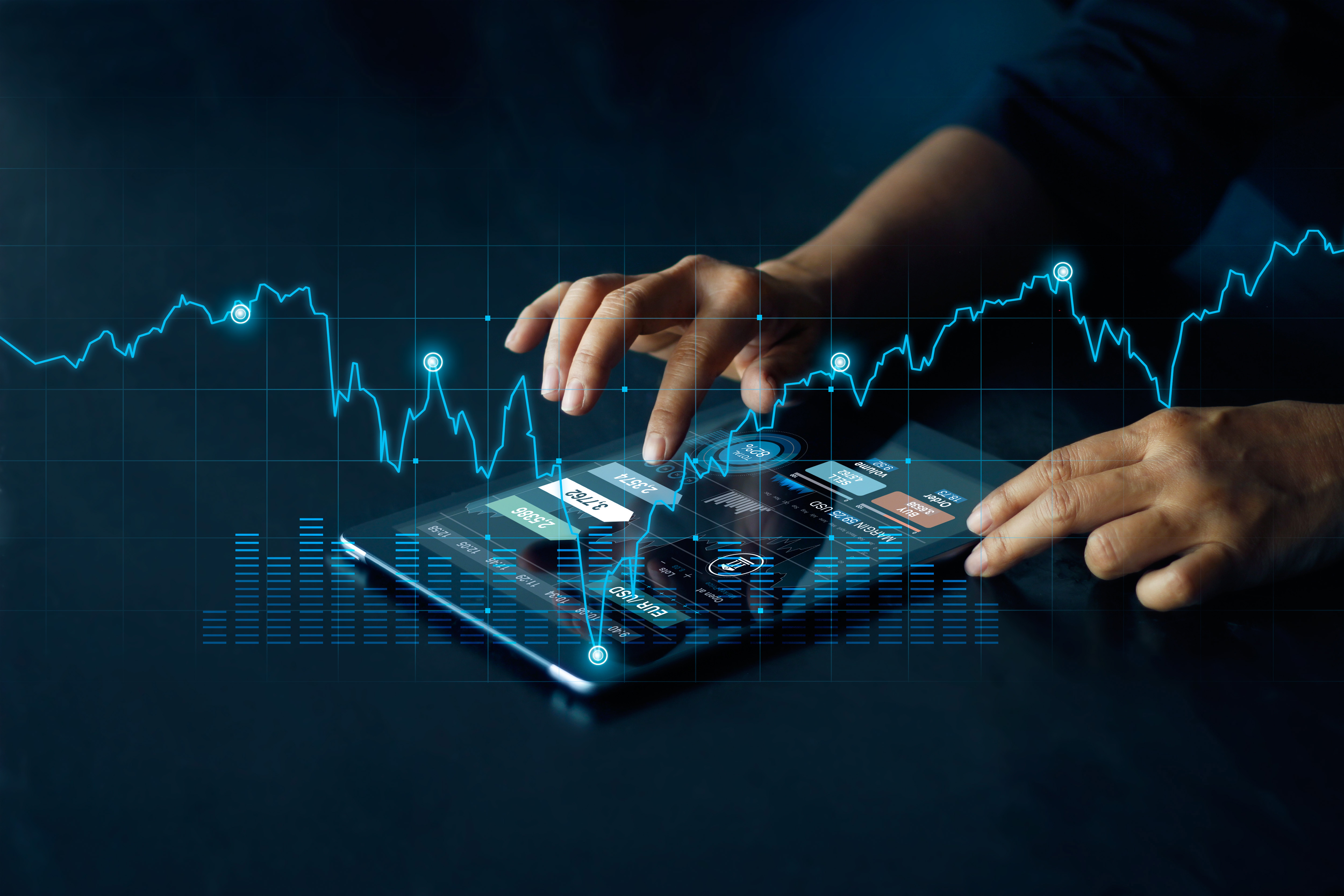
In an era of rapid technological advancement, the Banking and Financial Services sector stands at the forefront of innovation. As data permeates every facet of these industries, analytics has emerged as the linchpin driving strategic decision-making and operational efficiency. To maintain their competitiveness, banks must remain up to date with these recent analytics trends:
- AI and Augmented Analytics
- Composable Data Analytics
- Cloud-based Analytics and the Multi-cloud strategy
1. Augmented Analytics
Augmented data analytics is a term used to describe using artificial intelligence (AI) and machine learning (ML) technologies to enhance traditional data analytics in the banking and finance industry. It involves the integration of these technologies into existing data analytics systems, providing more accurate and timely insights that can inform business decisions. Gartner predicts that by the end of 2023, augmented data management will lessen the need for financial analysts for repetitive and regular data management duties, saving up to 20% of their time for collaboration, training, and high-value analytics tasks [1].
The integration of augmented data analytics into the realm of banking and finance heralds a transformative advantage: the expeditious and precise analysis of copious datasets. Data analytics often necessitate redundant manual efforts for sorting and interpreting information, rendering the process susceptible to errors. On the contrary, deploying Artificial Intelligence (AI) and Machine Learning (ML) technologies empowers banks and financial institutions to discern intricate trends and patterns within their datasets swiftly and with utmost precision. This imparts invaluable insights, affording them a heightened state of informed decision-making, thus optimizing their business strategies.
For instance, JPMorgan Chase & Co. has emerged as a leader in the adoption of augmented analytics, especially within the banking industry. In 2016, the bank launched its Emerging Opportunities Engine, which uses predictive algorithms to identify which clients are primed for issuing or selling equity based on an AI analysis of granular datasets. Emerging Opportunities has been highly successful at reducing costs and enhancing revenue while paving the way for more targeted promotional campaigns and improved customer satisfaction. Moreover, this technology has assisted JPMorgan Chase in reducing data processing time ahead of the proposal timeline.
Other banks, like Goldman Sachs, have since followed suit in creating like-minded engines, while JPMorgan Chase has accelerated its adoption of augmented analytics in other areas. As such, augmented analytics is expected to be recognized as a commanding driver in the field of Power BI, data science, ML platforms, and embedded analytics in the near future.
2. Composable Data Analytics
Composable analytics is the latest trend in business intelligence. It takes advantage of new technologies like machine learning, intelligent data management, and microservices to transform how companies use data. To simplify it, imagine composable data analytics as a massive social media platform where businesses can find and send friend requests to any new technologies they prefer and apply them directly to the existing system. This way, companies can update and add new technologies that leverage all the available tools without disrupting other applications. Composable analytics is the next level of business intelligence by playing a crucial role in inter-company data exchange and integrating different technologies.
According to Gartner analysts, by the end of 2023, 60% of firms will combine elements from three or more analytics solutions to create business apps that integrate data and link insights to actions [2]. With that being said, composable data analytics is becoming more prominent and widely welcomed as it allows businesses to quickly integrate with any new technology solutions that they may like. As such, implementing a flexible and scalable approach such as composable data analytics will significantly enhance agility and efficiency in data analysis without disrupting or making changes to the existing system. By simply adding in or removing a solution by will at any time, banking institutions can pilot new technology, evaluating whether it is suitable for their businesses/system or not.
More than that, composable analytics can benefit banking institutions due to the large and diverse nature of the data that banks typically handle. Banks often deal with various data types, including financial transactions, customer data, market trends, and regulatory requirements. This data can come from multiple sources, including internal systems, external partners, and public datasets. By allocating each technology solution to an exact analysis process, composable data analytics can significantly enhance overall workflow thanks to integrating multiple data analysis solutions within a single platform. Varo Bank is one prominent example of implementing composable data analytics. This financial institution has attracted four million new accounts in the 13 months since obtaining its US bank charter in July 2020. Behind its story is the improved agility from leveraging composable data analytics, allowing it to “compose” with different IT solutions quickly built from best-of-breed capabilities. By integrating AI and cloud-based analytical solutions within a single platform, Varo Bank has peaked at the highest revenue thanks to the convenience and cost-efficiency of the composable approach. It notably results in lower operational costs (Varo estimates it serves customers at 25% of traditional banks’ costs [3]), improving business margins and allowing for more competitive solutions to be launched, opening up opportunities with which other banks cannot compete.
3. Cloud-based Analytics and the Multi-cloud strategy
Cloud-based analytic platforms enable financial institutions to store and analyze large amounts of data in the cloud, providing them with greater flexibility and scalability than traditional on-premises analytics systems. Banks and financial institutions can gain real-time insights into their operations using cloud-based analytics platforms and make faster, more informed decisions.
For instance, Starling Bank - the first UK bank to launch mobile-only banking accounts - has implemented a cloud-based analytics solution named BigQuery by Google Cloud. To put it simply, BigQuery can be described as an integrated platform between cloud computing and low-code. Within this platform, data can be restored in the cloud to be accessed at any time. Employees then can use scripted programming code to analyze these data without having expertise in programming languages. BigQuery allows users to code new programs based on their suggestions or simply choose from their existing prompts. These prompts are continuously updated to meet any particular analytical need of the users. BigQuery, as such, has significantly reduced overall costs and manual efforts, enhancing employees' productivity. Moreover, BigQuery has also utilized the Google Maps Platform - a member of the Google Apps family - to improve the data-generating and analyzing process. Merchant identification and real-time notifications generated from Google Maps mean that analysts can easily determine who the customers have paid, when, and where.
With BigQuery driving its analytics, the bank has been able to see how its products are being used and respond to its customers' needs effectively, meaning that all product development is informed and prioritized based on customer behaviour. As a result, cloud-based analytical tools are predicted to become the leading solutions for a few more decades, especially when integrated with other cloud-based applications, significantly leveraging their already powerful usage.
Conclusion
The implementation of analytics in the banking and finance sector is undeniably upward, and this trend is poised for sustained growth in the years ahead. Remarkably, McKinsey forecasts that by enhancing analytics endeavours, the global banking industry could potentially witness a remarkable surge of up to $1 trillion in annual profits [4]. With the expanding troves of data and the dynamic innovation spearheaded by FinTech leaders like TreasurUp, the adoption of analytics is slated to become even more pivotal. This is especially pertinent for banks seeking to keep pace and maintain a competitive edge in the rapidly evolving landscape of finance.