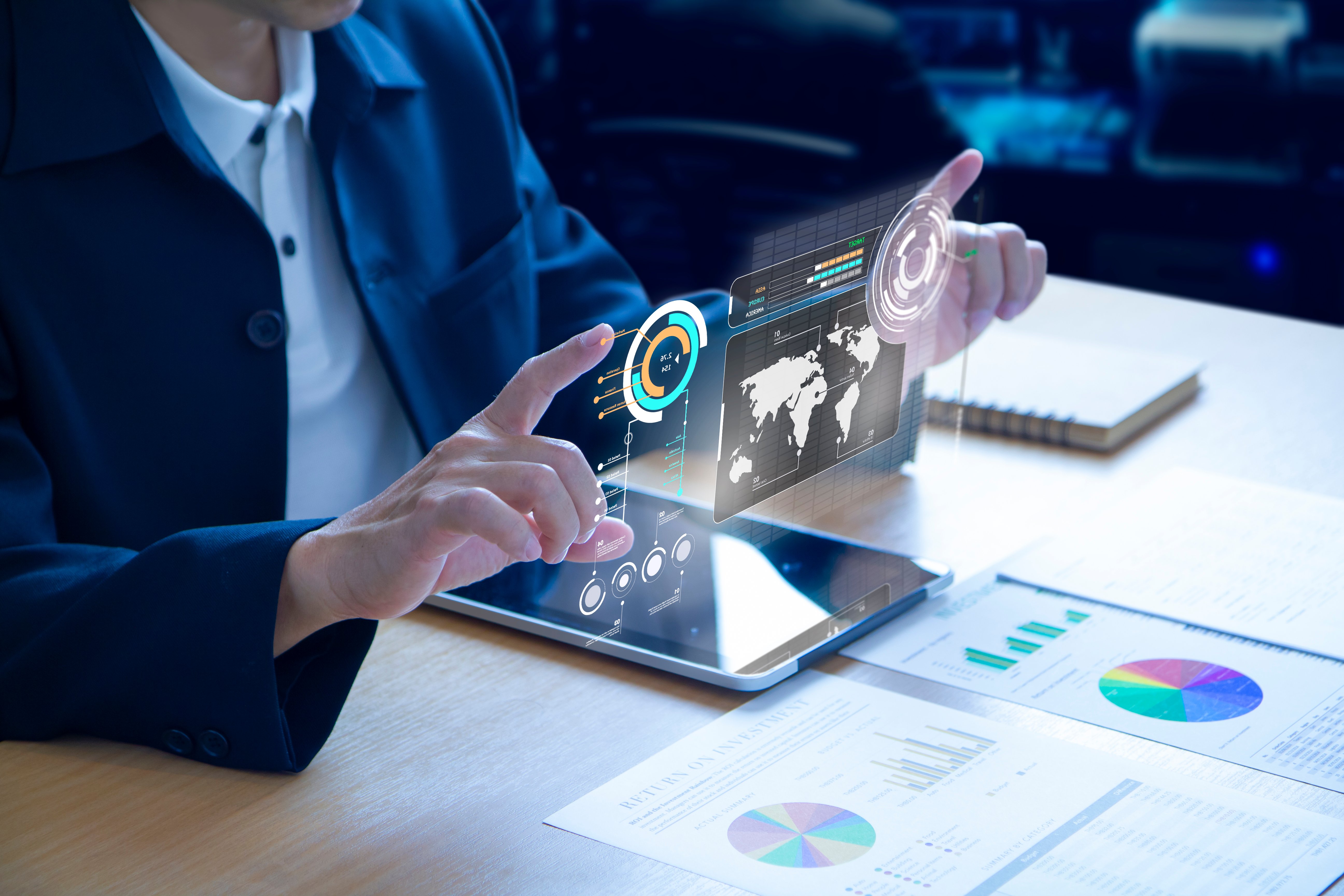
Artificial Intelligence (AI) applications are concentrated in any domain where data is available. Whenever and wherever real-life data is inaccessible, synthetic data promises to fill the gaps.
Synthetic data - the digital replica of real-life data
Synthetic data refers to a range of computer-generated data types that simulate original, real-life records. It can be created by stripping any personally identifiable information (such as names or license plates) from a genuine dataset, fully anonymous the data. Another creation tactic is to use the original dataset to build a generative model that generates data that bears high realistic quality.
A generative synthetic data model comes in three forms, namely GANs (Generative Adversarial Networks), VAEs (Variational AutoEncoders), and Autoregressive. While GAN models utilize a generative and discriminative network in a zero-sum game framework, VAE attempts to recreate output from the input using encoding and decoding methods. Autoregressive models train the network to create new individual pixels based on previously presented ones. In contrast to most data creation methods, which are static and require regular calibration, synthetic data is highly autonomous and easily controlled.
Driver for Artificial Intelligence 2.0
Over the last few years, there has been increasing concern about how biased datasets can lead to AI algorithms perpetuating systemic discrimination. Gartner predicts that through 2022, 85% of AI projects will deliver unsatisfactory outcomes due to bias in data, algorithms, or the teams responsible for managing them [1]. Synthetic data promises to deliver the advantages of AI without the downsides.
Synthetic data could become the saving grace for many big data applications for its data-anonymizing nature. Businesses can extract insights from such data without impacting privacy compliance. Synthetic data can add more variety and reduce bias by incorporating uncommon occurrences that indicate plausible scenarios that are difficult to find in original data.
Additionally, the cost of training an AI system is better optimized with synthetic data. When training models, developers frequently want big, precisely labeled datasets. Yet collecting and labeling these enormous datasets with thousands, or even millions of objects, is time-consuming and costly. In conjunction with AI training, synthetic data can achieve higher accuracy at a lower cost. For instance, a $5 training image obtained from a data labeling provider could be produced artificially for as low as $0.05.
Not without limitations
Synthetic data offers compelling benefits, but it is not easy to realize them. Here are some of the challenges that come with generating synthetic data:
- Realism—synthetic data must accurately reflect the original. However, business departments, customers, or auditors may also require assurances of privacy preservation. It can be challenging to generate realistic data that does not disclose actual private data. On the other hand, if the data is not highly precise, modeling efforts based on unrealistic data cannot generate valuable insights as they will not reflect the crucial patterns for the training or testing project.
- Bias—often creeps into ML models trained on artificially generated datasets. Both real-world and synthetic data may contain an inherent or historical bias. If the synthetic data accurately mimics the original, it can reproduce the same tendencies in the newly generated data. Data scientists must train the ML models to account for bias and ensure the synthetic dataset delivers impartiality.
- Privacy—If the original data contains personally identifiable information (PII), then the synthetic data generated may be subject to privacy protection regulations. Hence, business needs to make sure fair AI conditions and HIPAA compliance are thoroughly met before adopting synthetic data.
Synthetic data fuels critical industries
As synthetic data enables training AI systems in a completely virtual realm, it can be readily customized for various use cases ranging from healthcare and automotive to financial services.
- Healthcare: Data privacy seems to be the biggest roadblock on the path to innovation and more advanced AI applications. Synthetic patient data can improve machine learning/deep learning model accuracy by increasing the training dataset size without violating data privacy regulations.
- Automotive and Robotics: Research to develop autonomous gadgets such as robots, drones, and self-driving car simulations pioneered the use of synthetic data. This is because real-life testing of robotic systems is expensive and slow. Synthetic data enables companies to perform product testing in thousands of simulated scenarios at a lesser cost.
- Banking, Finance and Insurance: Fraud identification is a significant part of any financial service, even though fraudulent transactions are rare. With synthetic data, fraud detection methods can be tested and evaluated for their effectiveness. In addition, customer behavior can be studied in-depth through the analytics of synthetic customer transactions. This proves especially useful in an industry where data usage and customer privacy are particularly limiting.
Last year, McKinsey revealed that 49 percent of the highest-performing AI companies have already been using synthetic data to train their AI models [2]. Companies will be hearing a lot more about it in the coming years, yet what it takes to move forward is not only the right technologies but also the relevant skillset, framework, and metrics in the new AI 2.0 era.
Sources