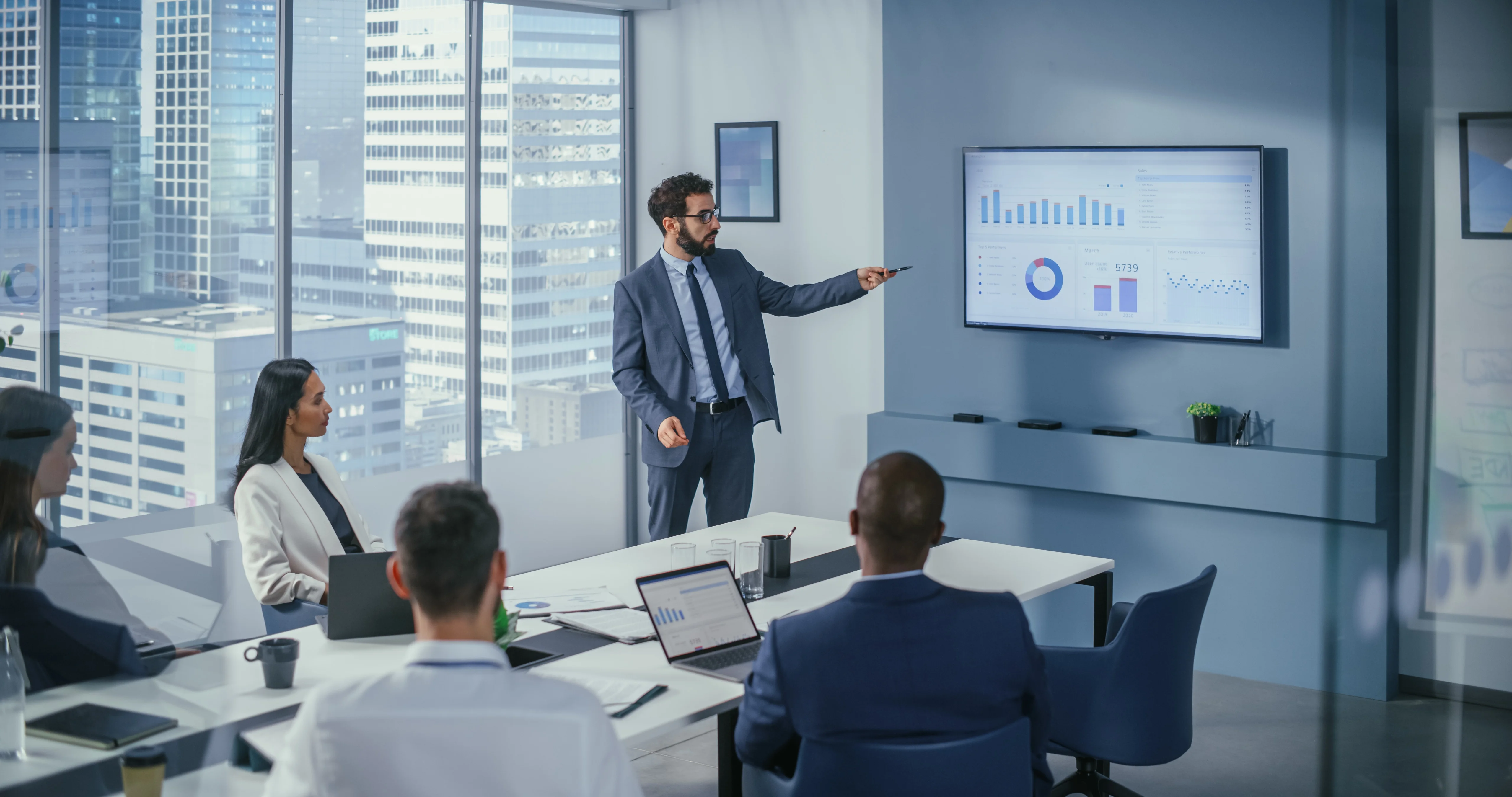
Nowadays, organizations are embarking on a journey to scale AI. Scaling AI is an approach to implement AI across the company, including establishing a continuous process to prioritize use cases, creating a decision framework, putting responsible AI at the forefront, and investing in data and AI literacy. However, successfully scaling AI involves navigating significant challenges related to data, tools, cross-team collaboration, and governance. By aligning these four key pillars, companies can set themselves up for success.
Prepare High-Quality Data
As data plays a central role in AI systems, scaling AI must rely on high-quality data. However, several challenges, such as errors, missing values, and bias, can prevent the AI model from developing and performing effectively. Additionally, poor data governance makes it difficult to access and integrate data from various sources, which can drive up costs and limit actionable insights. In fact, according to research by MIT Technology Review, 72% of technology executives believe that if their companies fail to meet AI goals, data issues will be the main culprit.
To ensure high-quality data over time, organizations need robust cleaning, validation, and continuous monitoring systems. Automated data validation processes, for example, can help detect anomalies and outliers to help the model maintain its accuracy. But what exactly is high-quality data? High-quality data is clean, accurate, consistent, and free from bias, which are the key attributes that allow businesses to harness the full potential of their data for improved decision-making and AI-driven results. The data must also be up-to-date to maintain the model's relevance and performance in dynamic environments.
Implement The Right Tools
When scaling AI within an organization, selecting the right tools is essential for aligning AI initiatives with broader business goals. This ensures AI efforts directly contribute to solving key challenges. According to IBM, providing environments where trainers can experiment with, develop, and scale AI models effectively is essential.
Building a single ML model can require various specialized systems, often assembled by data science practitioners from a wide variety of open-source and proprietary tools. This effort is called machine learning operations, or MLOps, and includes tools for building, maintaining, and monitoring AI and reporting on its outputs to internal stakeholders and regulators. MLOps establishes best practices and tools for AI development, deployment, and adaptability, while still maintaining speed and safety. Furthermore, MLOps allows organizations to navigate the complexities of scaling AI and ensures that AI systems remain adaptable to evolving market conditions, customer demands, or regulatory requirements.
Besides adopting MLOps, organizations should implement best practices in software engineering, such as using reusable code packages and modules. According to McKinsey, reusable code packages can expedite the development process and reduce costs. Specifically, these code packages can help reduce duplication and allow data teams to focus on strategic tasks instead of repetitive coding. With a more modular structure, AI/ML projects become leaner and more resource-efficient, making it easier to modify, expand, or repurpose AI projects. As 78% of the surveyed executives indicated that scaling AI and machine learning use cases to create business value is their top priority for enterprise data strategy over the next three years, organizations need to continuously improve AI/ML initiatives to meet evolving business needs.
Involve The Right People
Scaling AI requires the right combination of people. AI development requires multiple disciplines and stakeholders, including data scientists ( who design algorithms, build models, perform data analysis, and refine model features), ML engineers (who optimize and operationalize these models, ensuring they are scalable, production-ready, and capable of running efficiently across large datasets), software engineers, business leaders, and employees across the organization.
Most importantly, employees play a pivotal role in this transformation and are welcoming AI practices to improve productivity. A 2025 report by McKinsey revealed that while C-suite leaders estimate that only 4% of employees use generative AI for at least 30% of their daily work, employees themselves report this figure is 3 times higher. Thus, business leaders should invest in internal AI training programs, certifications, and university partnerships to develop talent pipelines, which are critical strategies for building an AI-ready workforce. Knowledge-sharing platforms can also encourage continuous learning and update employees with emerging AI trends.
Implement Strict AI Governance
AI governance is essential to ensure that businesses can scale AI effectively while maintaining safety and ethical standards. As AI becomes more integrated into business operations, it’s crucial to establish strong oversight to manage misuse and privacy violations. Specifically, a privacy breach in AI systems can occur when sensitive personal data is mishandled or exposed due to improper data management, inadequate security measures, or flaws in the AI model's design. For example, AI systems that process large amounts of personal information may share or leak data if proper safeguards aren’t in place. According to Gartner, more than 40% of AI-related data breaches will be caused by the improper use of generative AI by 2027. Additionally, if AI systems learn from or make predictions based on private information without consent, it can violate individuals' privacy rights and lead to legal consequences for businesses.
Thus, businesses can address these issues by investing in Responsible AI initiatives. These programs help companies stay ahead of potential risks, improve product quality, and ensure consistent ethical practices. According to PwC, 37% of U.S. enterprises that adopted Responsible AI strategies reported better AI management, reducing legal, financial, and reputational risks, and encouraging the responsible growth of AI.
AI governance also plays a key role in addressing human biases in AI development. AI systems are created by people and can inherit their creators’ biases, which can lead to harmful outcomes such as discrimination. For instance, in healthcare, algorithms have been found to disadvantage black patients by prioritizing cost over medical need. Therefore, a solid governance framework can help identify and correct these biases, ensuring that AI systems make fair and ethical decisions to protect human rights. A broad range of stakeholders, including developers, users, policymakers, and ethicists, must be involved in AI governance to ensure that AI systems are aligned with societal values. This helps build trust and accountability within organizations, regardless of any industries.
Co-create an AI-first Future with FPT
To scale AI successfully, enterprises need a trusted partner who can help cultivate an AI-first culture and strengthen the core pillars for scaling AI—access to the right data, advanced tools and frameworks, seamless collaboration among teams within organizations, and stringent data governance.
FPT, with a team of more than 1,000 AI engineers, integrates AI into all of our services and offerings to ensure the development of cutting-edge solutions. Recognizing the critical role of data in AI development, FPT emphasizes strong data compliance and adheres to international standards across all industries, including HIPAA, GDPR, and more. With a wide partnership network with global AI leaders, including NVIDIA, AITOMATIC, Mila Institute, and Landing AI, FPT is committed to driving innovation and excellence.
As a founding member of the AI Alliance, established by IBM and Meta, FPT continues to advocate for responsible AI practices on a global scale. By building a robust AI partner ecosystem with global technology pioneers, FPT is shaping the future of AI and advancing responsible innovations.