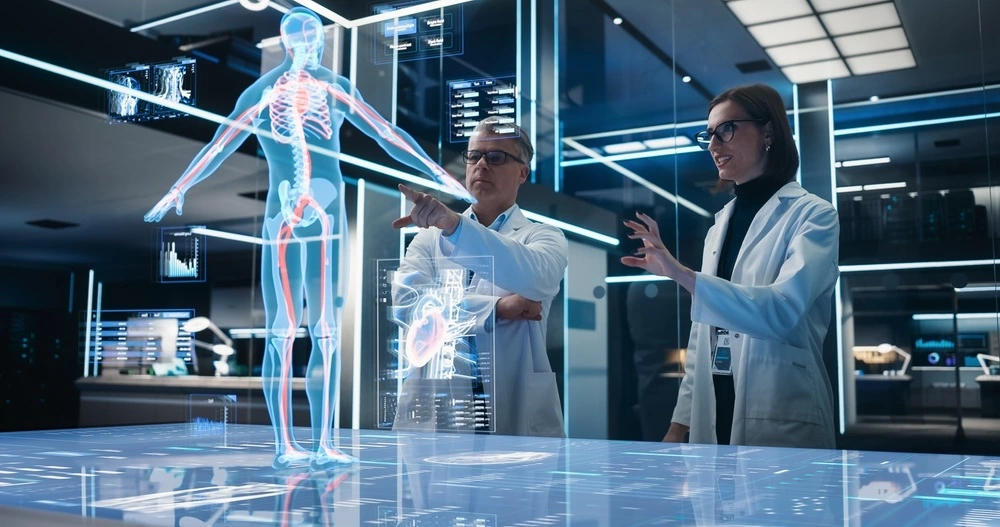
The emergence of artificial intelligence has brought various ethical concerns. Therefore, Responsible AI is an approach to developing and deploying artificial intelligence (AI) in a safe, trustworthy, and ethical fashion. As 94% of IT leaders believe more attention should be paid to responsible Al development, the healthcare industry needs to devise strategies to alleviate the current AI challenges.
Current challenges of AI in healthcare
Training data biasBias can stem from various reasons, one of which is how researchers and healthcare institutions collect and prepare their data to develop AI models. If the training data for an ML-based system has sampling bias, the patient cohort is not representative of the target population. For example, if an ML-based system recognizes skin diseases, such as melanoma, based on images of people with white skin, the ML system might misinterpret images from patients with darker skin tones and fail to diagnose melanoma due to sampling bias (Adamson & Smith, 2018). Despite representing only 1% of skin cancers, melanoma is responsible for over 80% of skin cancer deaths. As a result, ML-developers should disclose the training data details, including patient demographic and baseline characteristics such as age, race, ethnicity, and gender.
Algorithmic biasResearch by Panch et al. (2019) defines algorithmic bias as the application of an algorithm that includes inequalities in socioeconomic status, race, ethnic background, religion, gender, disability, or sexual orientation. If a dataset used to train an AI system lacks diversity, the AI may develop biased algorithms that perform well for certain demographic groups while failing others. This can aggravate current health inequities and lead to poor health outcomes for underrepresented groups. In 2023, UnitedHealth faced a class action lawsuit for illegally using an AI algorithm to deny rehabilitation care to seriously ill patients, even though the companies knew the algorithm had a high error rate.
AI biases can stem from training data and AI algorithms.
Lack explainability
Responsible AI implementation requires explainable AI, which involves incorporating fairness, model explainability, and accountability into AI methods real organizations use. In the healthcare industry, AI is usually utilized to predict sepsis or heart failure by analyzing extensive patient data, such as vital signs and lab results. However, these models often rely on complex calculations, particularly those using neural networks in deep learning, which can be difficult for humans to understand. This difficulty in understanding is known as the "black box" problem, referring to the challenge of comprehending how algorithms make specific conclusions. The nature of this "black box" model can pose challenges for medical professionals who want to fully leverage its predictions without understanding its rationale, particularly in life-or-death situations.
Misleading healthcare resultsAI hallucinations are incorrect or misleading results that AI models generate. These errors can be caused by various factors, including insufficient training data, incorrect assumptions made by the model, or biases in the data used to train the model. AI hallucinations can be a problem for AI systems that are used to make important decisions, such as medical diagnoses or financial trading. Given the rise of AI, one group of researchers carried out a study about ChatGPT to explore questions about their health and medication plans. The researchers found that ChatGPT often generates inaccurate, even dangerous, responses. In one question, the researchers asked ChatGPT whether the COVID-19 antiviral Paxlovid and the blood-pressure-lowering medication verapamil would react with each other in the body. ChatGPT responded that taking the two medications together would lead to no adverse effects. In reality, people who take both medications might have a large drop in blood pressure, which can cause dizziness and fainting.
What has been done?
AI regulations have been introduced to promote ethical AI implementations.
Promoting responsible use of AI in every institution that harnesses this innovative technology is essential. As new uses of AI in healthcare continue to grow, the need for development and evaluation standards becomes even more important to ensure ethical applications. In May 2024, healthcare leaders in the U.S. came together to introduce theTrustworthy & Responsible AI Network (TRAIN), a consortium created to explore and set standards for the safe application of AI in healthcare. TRAIN members potentially can help:
- Improve the quality and trustworthiness of AI by sharing best practices related to the use of AI in healthcare settings;
- Enable registration of AI used for clinical care or clinical operations through a secure online portal; and
- Provide tools to measure outcomes with AI implementation.
In another instance, the European Union has introduced the AI Act, a regulation focusing on the responsible use of AI. The regulation indicated that diversity, non-discrimination, and fairness are critical. This means that AI systems should be developed and used in a way that includes diverse actors and promotes equal access, gender equality, and cultural diversity while avoiding discriminatory impacts and unfair biases. Another important criterion is to evaluate and prevent the negative impact of AI on vulnerable individuals, including people with disabilities, and promote gender equality.
What still needs to be done?
Besides establishing stringent policies on AI, a multi-stakeholder approach is needed to ensure the implementation of responsible AI. AI development needs to involve diverse stakeholders such as developers, ethicists, policymakers, and patients to ensure a comprehensive approach and promote ethical AI implementations.
Additionally, responsible AI also calls for significant effort among healthcare researchers to find a solution to unbiased AI. One study by Obermeyer et al. (2019) found evidence of racial bias in one widely used algorithm. The algorithm utilized insurance claims data to forecast the future health requirements of patients based on their recent health expenses. However, the creators of the algorithm failed to consider that healthcare spending for black Americans is usually lower than that for white Americans with similar health conditions, not due to their health status but because of barriers to accessing healthcare, inadequate healthcare, or lack of insurance.
A mult-stakeholder approach is needed to promote responsible AI.
The researchers informed the manufacturer, who conducted tests using its data to confirm the problem and collaborated with the researchers to mitigate the bias in the algorithm. After developing new predictive algorithms to predict the outcomes, the researchers reduced the number of excess active chronic conditions among black people and achieved an astounding 84% reduction in sampling bias. These results suggest that label biases are fixable, and healthcare researchers must change the data labels fed to the algorithm.
Notably, technology organizations are joining hands to promote responsible AI in business operations. For instance, FPT Software has strategically partnered with Mila Institute to collaborate on research projects on large language models (LLMs) and natural language processing (NLP) while promoting Responsible AI. The company has also partnered with NVIDIA and Landing AI to amplify Vietnamese skills and expertise, position Vietnam as a global AI hub, and accelerate AI applications across various industries globally.
Empowering healthcare with ethics and transparency
With the rapid growth of AI, it is crucial for not only healthcare but also other sectors to be aware of the challenges. Therefore, healthcare institutions must focus on obtaining high-quality data, including thoroughly analyzing the training data to confirm that it is representative and suitable for creating unbiased AI models. Before starting AI software development projects, businesses should perform ethical impact assessments to recognize potential ethical implications. It is also crucial to establish transparency in AI to help users understand how decisions are reached. Most importantly, AI developers should adopt a user-centric approach to AI software development ethics by involving various stakeholders in the creation of AI systems. As per Gartner's prediction, 50% of governments globally will enforce responsible AI through policies by 2026. Therefore, adhering to AI regulations is essential to secure a safer future in healthcare.