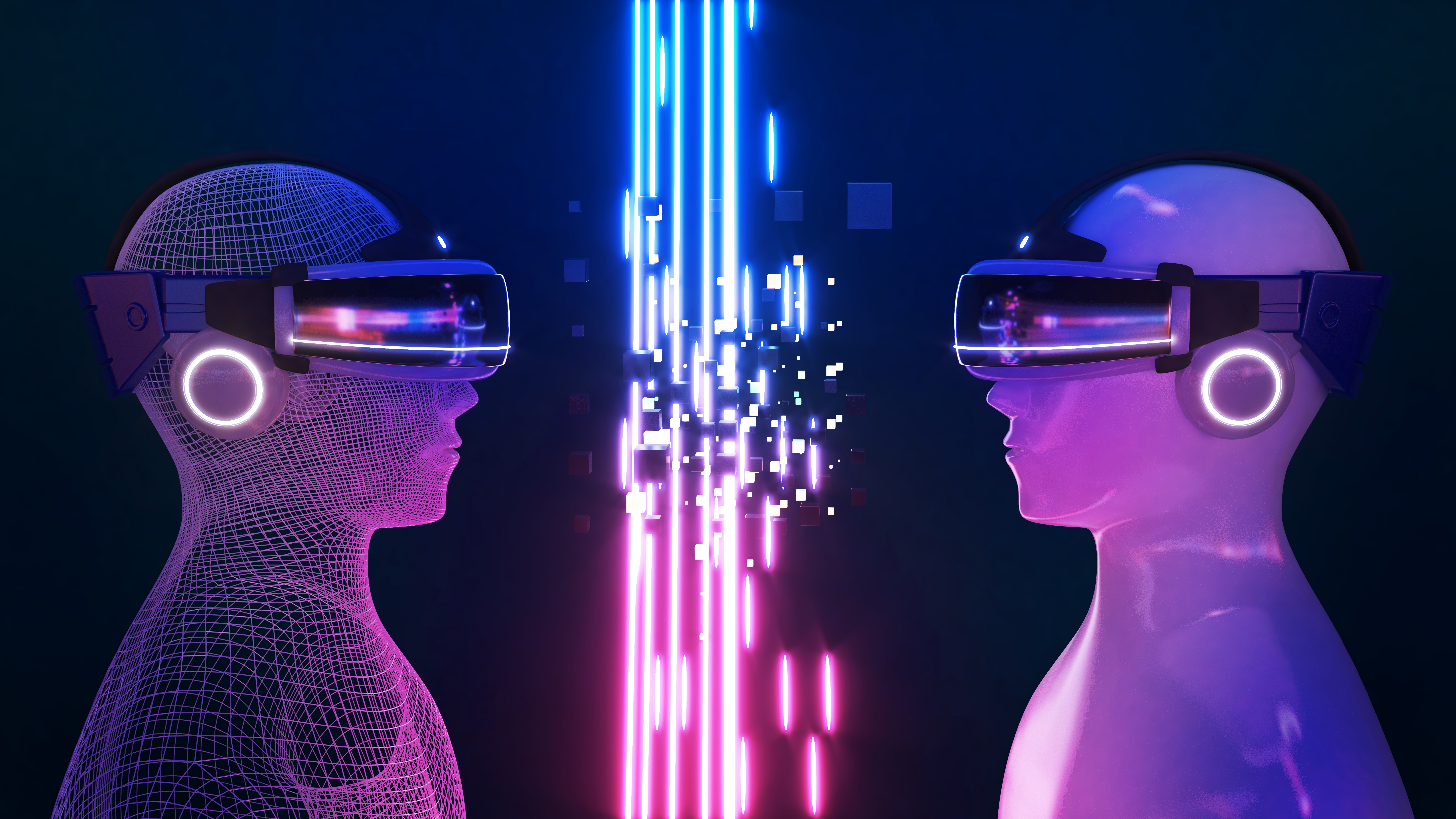
AI-driven cyberattacks take on diverse forms, posing potentially severe consequences. A recent survey by Forrester revealed that 88% of security experts anticipate the mainstream adoption of AI-driven attacks – and it is only a matter of time [1]. A groundbreaking approach is pitting AI-powered solutions against AI-powered threats.
Criminal minds – Understanding how AI is exploited
Through machine learning techniques, such as reinforcement learning and generative adversarial networks, cybercriminals can devise advanced cyberattacks capable of breaching cybersecurity. Thus, businesses should watch out for cybercriminals’ tactics in exploiting AI, including:
- Crafting more intricate malware: Generative AI enables the creation of malware strains. These malware strains often incorporate evasive techniques, such as polymorphic code, which continually changes its appearance, or obfuscation methods that encrypt the malware's core code. This allows them to remain undetected by antivirus software and security protocols, allowing cybercriminals to steal sensitive data or launch cyberattacks before their harmful actions are exposed.
- Generating deep fake content: Generative AI's ability to create authentic-seeming imitations of human behavior, including text, speech, and images, facilitates fraudulent activities like identity theft, financial fraud, and the dissemination of disinformation.
- Overcoming CAPTCHAs and password guessing: Cybercriminals can now bypass security measures such as CAPTCHAs, commonly used to thwart unauthorized access by bots, with the assistance of machine learning. ML empowers hackers to perform repetitive tasks like password guessing and brute-force attacks.
- Sabotaging ML-based cyber threat detection: By inundating a security system with a multitude of false positives, hackers can exploit vulnerabilities and catch it off guard with an actual cyberattack.
New AI threats are on the rise
Acoustic side channel attack - When your keystrokes become hackers' target
Acoustic side channel attack (ASCA), initially studied in the early 2000s, has recently resurfaced as a significant concern. This revival is attributed to the surge in video conferencing, remote work in public places, and advancements in neural networks. A team of researchers from British universities has trained a deep learning model that can steal data from keyboard keystrokes recorded using a microphone. Their results were striking: a 93% accuracy rate when trained on Zoom keystrokes and a 95% accuracy rate when using a smartphone. Such an attack can severely impact the target's data security, as it could leak businesses’ sensitive information to malicious third parties.
The researchers offer several practical suggestions to address concerns regarding acoustic side-channel attacks. Users who are particularly concerned can consider implementing biometric authentication and utilizing password managers to minimize the need for manual input of sensitive information. Additional defense strategies include utilizing software replicating keystroke sounds, introducing white noise, or employing software-based keystroke audio filters.
Voice cloning
A new type of fraud has emerged, utilizing AI voice technology called 'voice cloning.' This method allows cybercriminals to create fake audio recordings or voice commands closely mimicking an individual's real voice. The consequences are alarming, including identity theft, deceptive phone conversations, and the spread of phishing emails. Sadly, this advancement has already claimed its first victim, a UK-based energy company that lost EUR 220,000 in a fraudulent transaction [2].
In this case, the CEO of the UK-based energy firm fell for a scam in which an AI-powered deep fake imitated the voice of the CEO of the company's German parent company. The fraudster used AI voice technology to replicate the CEO's superior's accent in phone calls, convincing the victim to transfer funds to a Hungarian supplier's account. The CEO complied with the initial payment request, but suspicions arose when the scammer demanded a second transfer. The stolen money was eventually moved to a Mexican bank account and dispersed to various locations.
AI vs. AI – Who will win?
As cyberattacks become more sophisticated and their consequences more serious, traditional security systems will become things of the bygone era. According to Forbes, 76% of enterprises have prioritized AI and machine learning in their IT budgets [3]. This trend is driven by the increasing volume of data that needs to be analyzed to identify and mitigate cyber threats, among other reasons. With the ability to learn from previous attacks and adapt, AI is an invaluable asset for cybercriminals and their adversaries.
The use of AI in cybersecurity has some serious advantages, such as faster threat detection, identification, and threat response. AI algorithms can analyze network traffic to identify patterns that indicate a potential cyber threat. AI can detect anomalies, unusual traffic patterns, or suspicious behaviors that may go unnoticed by human analysts by processing large volumes of network data. For instance, AI algorithms can identify communication with known malicious IP addresses, detect port scanning activities, or recognize unauthorized data exfiltration attempts.
The accuracy of AI in cybersecurity is further amplified by its ability to learn and adapt continuously. Machine learning algorithms can be trained on vast datasets that encompass diverse threat scenarios and behaviors, enabling them to improve their detection capabilities over time. As AI algorithms learn from new data, they can refine their models and identify emerging threat patterns with increased accuracy.
Most importantly, fostering collaboration between human experts and AI systems is essential. Human analysts can provide contextual understanding, finetune AI models' responses to emerging threats, and guide investment plans in robust cybersecurity measures. According to research, 82% of IT decision-makers plan to invest in AI-driven cybersecurity in the next two years, and 48% plan to invest before the end of 2023 [4].
Therefore, businesses need to map out the right strategies to train their AI models to defend against cyber threats by AI, such as:
- Anomaly detection: Develop AI models focused on anomaly detection. These models can identify unusual patterns or deviations from normal behavior, often indicative of AI-driven attacks.
- Behavioral analysis: Train AI to analyze and understand the behavioral patterns of AI-driven attacks. This includes recognizing patterns in malicious AI's interactions with systems, such as rapid, automated data scraping or unusual access patterns.
- Simulation and red teaming: Employ simulated attack scenarios and red teaming exercises to train AI in recognizing AI-generated threats. These exercises help AI systems adapt to the tactics and techniques used by malicious AI.
- Threat intelligence integration: Integrate threat intelligence feeds into AI models to update them with the latest threat information. This real-time data enhances the AI's ability to detect and respond to new threats.
- Adversarial training: Train AI models using adversarial techniques, exposing them to AI-generated attacks in a controlled environment. This helps AI systems learn to recognize and defend against such attacks effectively.
Letting AI meet its match
Businesses have long grappled with the persistent challenge of cybersecurity, and the advent of AI has further exacerbated the issue. Nevertheless, the progressive approach involves harnessing AI to identify anomalies and confront AI-driven security threats. Above all, the effective collaboration between humans and AI will be essential for training and maximizing AI's effectiveness in cybersecurity efforts.