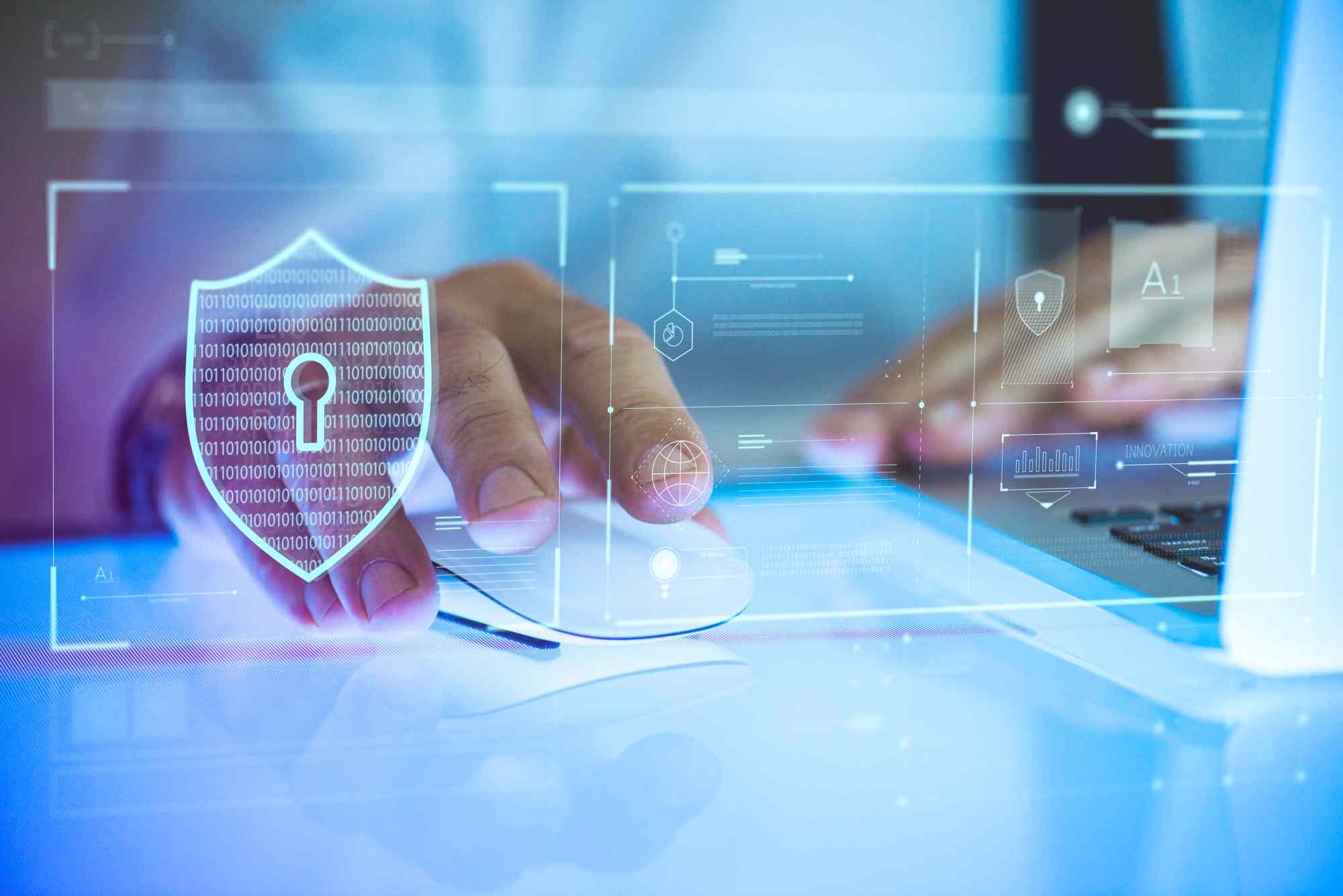
AI bias
Computer programs, in general, and AI, in particular, have long been praised for being a counter-attacking force of human bias, yet studies and real-life examples have shown otherwise. Humans naturally have cognitive bias, which is the tendency to simplify information processing based on individual preferences and experiences, bypassing logical reasoning. These biases are projected onto AI and computer programs as humans develop and train algorithms, and even more so when the technology’s performance is matched against humans’ capabilities. This issue can be traced back to as early as the 1900s when a British medical school was found guilty of discrimination. The institution used a computer program to identify applicants for interviews, which was developed to match human admissions’ decisions with up to 95% accuracy. Yet, the solution was later determined to discriminate against women and applicants with non-European names [2].
Even when AI overcomes the cognitive bias inherited from human developers, biased analysis is still inevitable if the input is already skewed. Training data can be biased in several ways, including historical data that reflects human biases and social injustice, and insufficient data that underrepresents minorities. Utilizing this information as input for AI will not only fail to cure human biases but also intensify them at scale and in turn, mislead decision-makers. For instance, Amazon secretly scrapped an AI recruiting tool after determining it to be gender discriminatory. The tool was built to automatically review and rate candidates’ CVs, trained on the company’s submitted CVs in the last 10 years, which mostly came from male applicants, given the tech industry’s male dominance. As a result, the algorithm learned to prefer male candidates and downgrade CVs that belonged to all-women college graduates or resumes that included phrases like "women’s” [3]. Similarly, racial bias was found in one widely used algorithm by the US health system, which predicts patients’ needs for extra healthcare. Using the healthcare spending as training data, which shows less spending on black patients than their white counterparts with similar risk levels, the algorithm mistakenly reduced the number of black patients identified for extra care by more than half [4].
Data privacy
Data plays an indispensable role in AI development, so much so that it becomes a make-or-break factor of AI’s performance. Indeed, only a continuous stream of massive and high-quality data can empower a high-performing AI analysis. Although technological advancement has enabled effortless data collection and storage, challenges persist regarding how organizations access and utilize said data for AI training. Despite the urgency, resolving these challenges should not come at the cost of the consumers’ data privacy. Prioritizing AI performance over safeguarding consumers’ data would lead to reputational damages as most consumers oppose having their data accessed without consent, even for personalization benefits such as targeted marketing, as cited by 67% of US consumers. More than that, violating consumers’ data privacy could bear legal risks as consumers advocate for more regulations to safeguard their data. Indeed, a survey by Prosper Insights & Analytics reveals that over 63% of customers expect new legislation that prohibits social media sites and search engines from selling their data [5].
Failing to comply with data privacy regulations has led companies to face lawsuits and restrictions in operations. For instance, in 2023, ChatGPT, a Gen AI-powered chatbot, faced a temporary ban imposed by the Italian data protection authority on the grounds that the company unlawfully collected users’ data and failed to implement an age-verification system [6]. In other news, Stability AI was sued by Getty Images for misusing over 12 million photos owned by the stock photo provider to train its Stable Diffusion AI image-generation system [6]. Similarly, artists worldwide have been taking AI companies to court, with one prominent case involving a group of seven artists filing a copyright lawsuit against Stability, Midjourney, DeviantArt, and Runway AI, for misusing their works to train Generative AI systems [7].
The rising data privacy concerns have drawn attention from legislative authorities, prompting a global investigation, research, and development of AI-related regulations. Following a temporary ban in Italy in March 2023, though later lifted, OpenAI continued receiving warnings from the Italian privacy authority over concerns that its ChatGPT breaches data protection regulations. Several data protection regulators, including Germany, France, and Spain, are following suit, launching investigations into ChatGPT’s data protection practices after receiving complaints about the application’s lack thereof. These incidents have accelerated the research and development of regulations relating to AI governance, with authorities including the European Union, South Korea, China, Canada, and Brazil leading the effort [8].
Data breach
Ignited by ChatGPT, Gen AI applications have received soaring popularity among business users for their capabilities to significantly enhance productivity. The possibilities enabled by Gen AI are boundless, ranging from artifact creation to code generation, bug identification and fixing. Yet, without proper security measures, Gen AI applications could expose organizations’ vulnerabilities, prominently including data breach risks. Indeed, a study has been conducted on AI-related data breach incidents, which estimated source code to be the most frequently exposed type of sensitive data, with an average of 158 monthly incidents for every 10,000 users. Other sensitive data that experience frequent breaches include regulated data such as financial, healthcare, or other personally identifiable information (18 incidents per month per 10,000 users) and intellectual property (4 incidents per month per 10,000 users) [9].
Unsurprisingly, global companies have developed controlling measures targeting the use of Gen AI at work. For instance, Samsung, the South Korean conglomerate, decided to prohibit ChatGPT use following an incident where an employee accidentally uploaded sensitive code to the platform [10]. Other well-known companies, including Apple, Spotify, and Verizon, have also banned or restricted employees from using Gen AI tools at work, over fears of jeopardizing company information and customer data [11]. Industry-wise, highly regulated sectors, including financial services and healthcare, take a more conservative approach with nearly 1 in 5 organizations completely banning the use of ChatGPT. Other sectors, such as technology, are embracing more flexibility in allowing employees to use Gen AI at work, as 1 in 4 technology companies use DLP measures to identify sensitive data uploaded on ChatGPT. Another measure involves real-time user coaching that frequently reminds employees of company policy and risks associated with Gen AI tools, implemented by 1 in 5 technology companies [9].
A global joint effort
With AI complexity soaring as it grows, potential risks will unquestionably increase at a corresponding rate, so much so that several tech leaders have called for a halt in AI development, fearing it could pose “profound risks to society” [12]. Thus, navigating AI risks will surely be challenging, and one viable solution would be developing robust AI governance practices. Yet, doing so requires tremendous efforts and investments, prompting a global collaboration in AI research and development. With multiple stakeholders joining hands in this regard, notably the AI Alliance consisting of organizations such as Meta, IBM, and FPT Software, fruitful results from research and development in AI governance are undoubtedly underway.