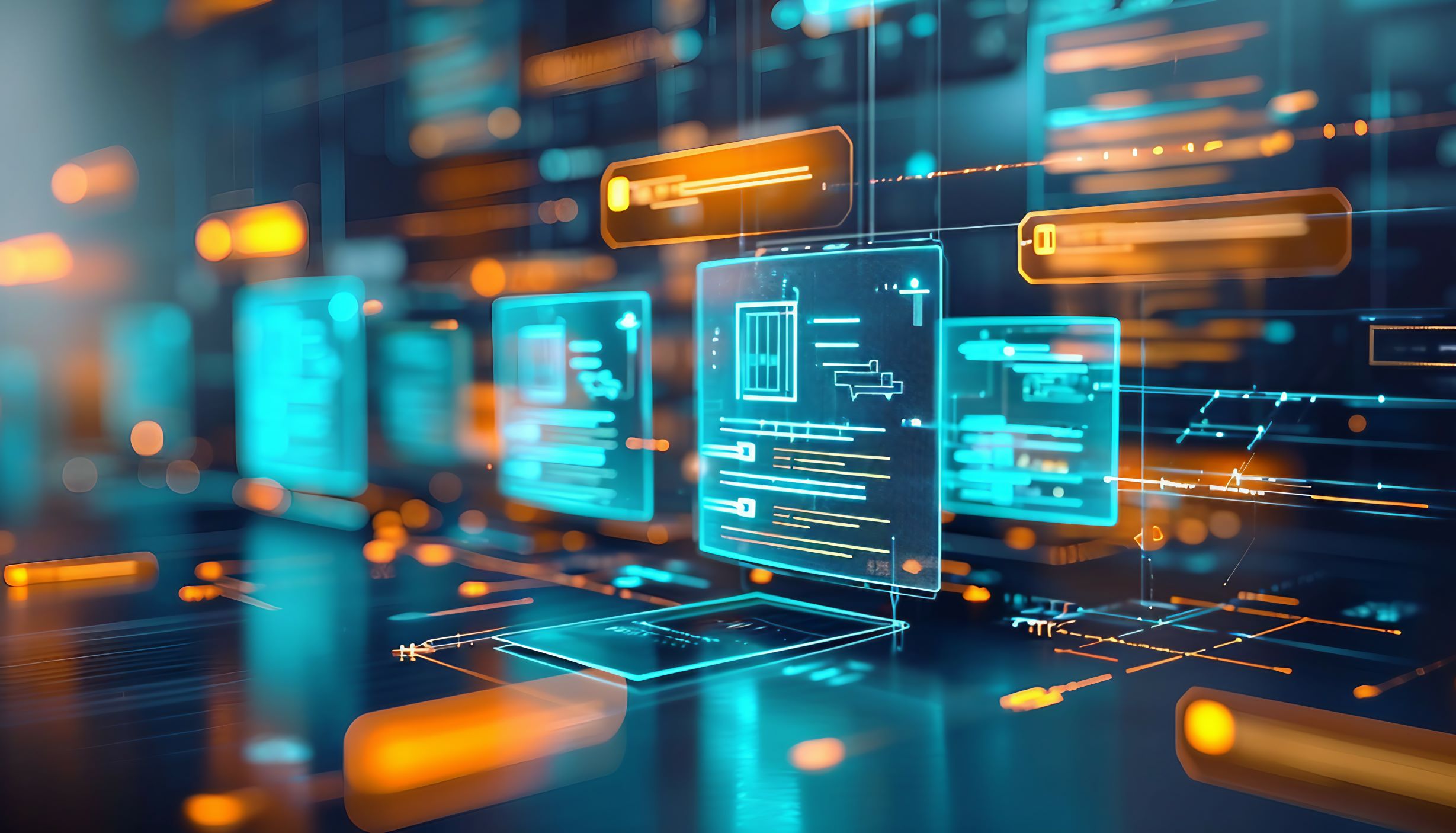
In the rapidly evolving business landscape, Artificial Intelligence (AI) has become a beacon of innovation and efficiency. Although AI's potential is widely recognized, the crucial role of data governance in its success is often overlooked. Effective data governance practices are essential, ensuring data is accessible, secure, and of high quality. This sets the foundation for successful AI implementation. Without proper governance, AI projects may suffer from flawed data, significantly blocking progress.
The critical role of Data Governance
The success of any AI initiative heavily relies on high-quality data. Data governance goes beyond simple management; it involves keeping data clean, organized, and securely accessible. This solid foundation is crucial for training AI models and deriving meaningful insights. Businesses that prioritize data governance are more likely to succeed in their AI efforts. For example, a retail company that used AI for personalized marketing significantly improved customer engagement by maintaining high data quality, demonstrating the direct impact of effective data governance.
Building a robust Data Governance framework
A comprehensive data governance strategy is key. This strategy begins with organizational recognition of data governance's importance and alignment on its objectives. Key components of an effective framework include:
- Stakeholder Engagement: Ensure all stakeholders understand and support the value of data governance.
- Initial Assessment: Review your current data governance maturity to identify areas for improvement and establish strategic plan (3 years) as well as tactical plan to be completed in 1 year.
- Tools and Best Practices: Use accelerators and tools to streamline the governance process, applying industry best practices for efficiency.
- Collaborative Approach: Promote a culture of collaboration between IT and business units, treating data governance as a joint responsibility.
Providing detailed guidance, such as specific assessment tools or frameworks, offers readers practical steps for each component.
Navigating Data Governance challenges
- Implementing data governance and integrating AI into business operations come with their own set of challenges.
- Organizations often encounter difficulties in aligning stakeholders with the definition and value of data governance, and understanding how it aligns with the company’s strategy.
- Being a member of a Data Driven Culture requires having relevant competencies. Organizations setting out to establish a Data-Driven culture often fail to recognize the need to train employees and to continuously adapt competencies to the changing conditions of technology and company strategy.
- Data governance and data management tools are often the first “implementation” of a data governance program. However, organizations frequently lack the data architecture strategy and policies to support the use of these tools.
- Data Governance is the practice that governs and maintains an organization’s data culture, requiring collaboration from everyone in the organization. Yet, organizations often misunderstand the level and type of investment needed for a sustainable and adaptable data governance program. As a result, investment is usually made only for the initial changes and not for ongoing business support, leading to a loss of momentum after the project is completed or the initial proponents move on.
To navigate these challenges, businesses must embrace a collaborative approach that involves both IT and business units in the governance process. This cooperation ensures that data strategies are in harmony with business goals and that the governance framework aligns with the organization's broader vision. Starting small and scaling up allows companies to learn from early implementations, refine their strategies, and progressively develop a strong data governance and AI ecosystem. Investing in implementation and organizational change is essential, but it's also important to set aside a budget for the practice of data culture going forward.
Initiating the Data Governance Journey
A structured approach to the data governance journey is essential for leveraging data as a strategic asset unlocking AI’s potential. It ensures comprehensive and aligned efforts across the organization, facilitating clear planning and execution.
Beginning the data governance journey entails a methodical approach:
- Stakeholder Alignment: Secure consensus on the importance of data governance for risk mitigation and seizing business opportunities.
- Assessment: Evaluate the organization's data strategy, maturity, and governance framework.
- Roadmap: Develop an implementation plan emphasizing gradual improvement.
- Execution: Implement the plan in stages, focusing on early successes to encourage broader adoption.
This journey should be marked by continuous evaluation and adjustment, ensuring that the data governance strategy remains aligned with evolving business needs and technological advancements.
Cost considerations
Embarking on data governance does not necessarily involve high costs. Typically, expenses range from moderate to substantial, emphasizing gradual enhancements and long-term sustainability over immediate, high-cost implementations. Strategic investments in technology, personnel training, and process optimization can yield significant returns by preventing costly data mismanagement incidents and enabling more efficient operations.
In essence, mastering data governance is crucial for leveraging the full potential of AI implementation in business. By establishing strong governance practices, companies can ensure high-quality data availability for training AI models generating meaningful insights and securing a competitive advantage. Through strategic planning, collaborative efforts across IT and business sectors, and dedication to ongoing improvement, organizations can navigate the intricacies of data governance and harness its benefits in the age of AI-driven transformation.