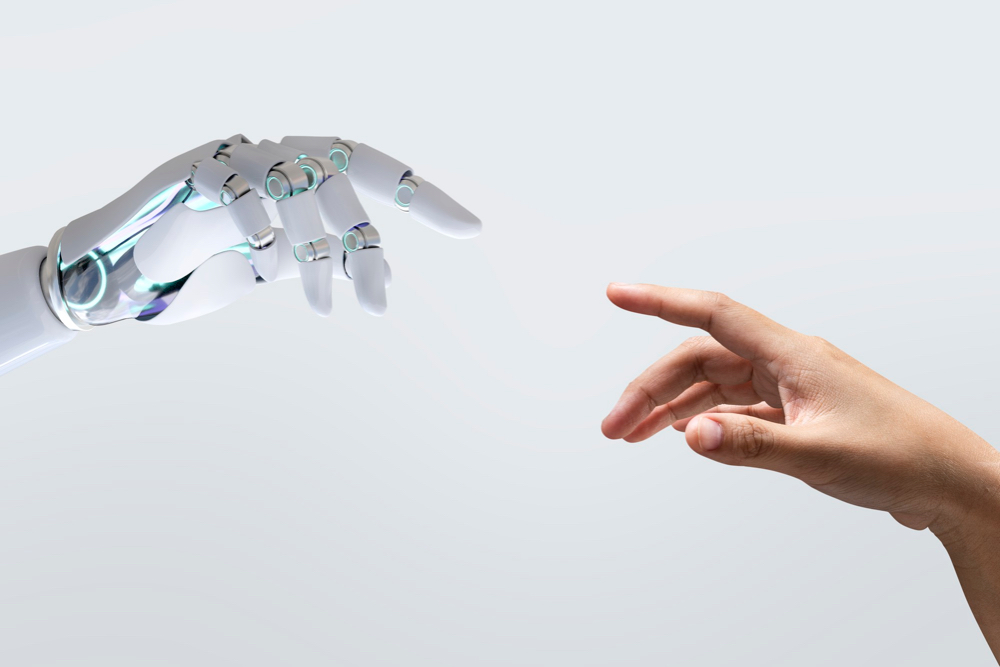
In the dynamic technology landscape, AI is no longer a trend but a critical need to gain a competitive edge. As AI continues to progress, the tech industry is now shifting its focus towards Large Vision Models (LVMs), which aims to transform visual recognition and analysis across various industries, such as healthcare and automotive.
What is Large Vision Model?
A "large vision model" (LVM) is a cutting-edge artificial intelligence model designed for visual tasks, such as image recognition, object detection, segmentation, and image creation.
Similar to how Large Language Models deal with text, LVMs based on Vision Transformers, are trained on massive datasets of images and videos to learn how to recognize patterns, classify objects, and even generate new visual content. Vision Transformer architectures facilitate LVMs to generalize effectively knowledge in massive training data. In turn, LVMs offer superior performance in few-shot and zero-shot inference on a variety of downstream tasks.
Applications of Large Vision Model
Large Vision Model in Healthcare
Healthcare providers usually face difficulty detecting subtle abnormalities, as human anatomical structures can exhibit intricate shapes and textures. Thus, large vision models are revolutionizing medical imaging in healthcare with:
- Image reconstruction and synthesis: Healthcare providers often store medical imaging data, such as MRI and CT scans, in unstructured formats. To make sense of this data, they need to reconstruct it to retrieve clear images without losing details. Traditionally, this process is time-consuming due to complex algorithms. Therefore, vision transformers have significantly improved image reconstructions. Vision transformers improve image reconstruction through tokenization – the process of dividing the image into smaller patches treated as tokens. By effectively focusing on relevant parts of the image and prioritizing crucial features during reconstruction, vision transformers ensure the retention of important details within seconds.
- Image segmentation: Research by Chen et al. (2021) proposed the TransUNet model – one of the earliest innovations – which combines Vision Transformers (ViT) with the UNet architecture for medical imaging segmentation. TransUNet combines the strengths of Unet and Vision Transformers. UNet can improve object segmentation and preserve fine details yet struggles with processing sequence-to-sequence frames and extracting features within an image. ViT, on the other hand, can handle sequence-to-sequence features but lacks feature localization. Therefore, TransUNet made image segmentation effective for multiorgan segmentation, which is crucial in analyzing complex structures in MRI and CT images.
- Surgical scene reconstruction: In surgical settings, ViT-based stereo transformers reconstruct dynamic surgical scenes, which are vital for surgical education, robotic guidance, and context-aware representation. A study by Wang et al. (2021) used a Swin Transformer – a vision transformer model – to accurately reconstruct sinograms from CT scans, producing high-quality images, reducing radiation doses, and enabling early cancer detection.
- Medical report generation: Utilizing vision transformer technology can assist healthcare providers in creating radiology reports, surgical instructions, and other clinical reports globally by retrieving vast amounts of information stored in health information systems. Vision transformer technology effectively addresses the challenges of managing biased medical data and long, inconsistent paragraphs. You et al. (2021) used the AlignTransformer framework to produce a long, descriptive, and coherent paragraph based on the analysis of medical images. The framework functions in two stages: first, it aligns medical tags with the related medical images to extract features, and then it uses the extracted features to generate a long report based on the training data for each medical tag.
Acknowledging the power of computer vision, FPT Software strategically partnered with Landing AI - a leading computer vision and AI software company based in the United States – to leverage the power of computer vision. In the “Visionary Integration: Showcasing the Future of Computer Vision” workshop in March 2024, FPT Software’s Chief Artificial Intelligence Officer Nguyen Xuan Phong reinforced the importance of computer vision integration into FPT Software’s ecosystem. He indicated: “Landing AI possesses the world's most advanced Computer Vision technology (transformer-based architecture) with extensive compatibility. In the future, we need to harness the power of computer vision across operations to reap the most values for FPT.” FPT has also experimented with the application of Landing AI in the healthcare sector and achieved promising results. Landing AI supported diagnosing 30 dermatological diseases with a model trained from 8,844 images, achieving an accuracy rate of 93%. Landing AI's current imaging technology has enhanced its diagnosis capabilities by 10 times compared to previous imaging technology.
Large Vision Model in Automotive
In the automotive industry, advanced vision models are crucial in enhancing driver assistance systems to ensure safer driving experiences. A key task within these systems is detecting and classifying vehicles, essential for Advanced Driver Assistance Systems (ADAS) and Intelligent Transportation Systems (ITS), which aim to reduce road accidents and save lives. According to the World Health Organization (WHO), approximately 1.19 million lives are lost due to road traffic crashes annually, with millions more sustaining non-fatal injuries and disabilities.
Addressing this pressing issue, research by Taki and Zemmouri (2023) proposed an innovative solution to enhance safety by leveraging a vision transformer model. Specifically, they utilized the Pre-Trained Model of Vision Transformer, the latest advancement in computer vision, to tackle the vehicle classification problem. Traditional object detection models often struggle under challenging conditions such as low-quality images, nighttime, and insufficient illumination. To overcome this, the researchers utilized the ImageNet-21k dataset, which included 4800 tiny, low-resolution vehicle images categorized into six classes: Bike, Car, Juggernaut, Minibus, Pickup, and Truck. The study achieved promising results with an accuracy of 99.3% with a vision transformer, surpassing the previous approaches in vehicle classification tasks.
In another case, FPT Software utilized Landing AI’s solutions to help a prominent car interior supplier control the quality of assembling car doors. The solution was deployed in just one month, from collecting data to labeling, training, and deploying the model. Thanks tthe company achieved 99.7% accuracy and optimized quality control time, reducing from 3 minutes to 2 seconds.
Large Vision Model – Enlightening the vision of tech landscape
The integration of large vision models with existing Large Language Models is becoming increasingly crucial as the technology industry evolves. This combination has enabled the creation of comprehensive AI systems that can navigate and understand both textual and visual information seamlessly. It also facilitates the smart way for human interacting with AI systems naturally by either text or voice.
With over a decade of AI investment, FPT Software has achieved significant milestones. With an AI ecosystem with over 20 products and solutions, serving more than 20 million users in 15 countries, FPT Software continues to harness the power of computer vision across all industries in the long run.