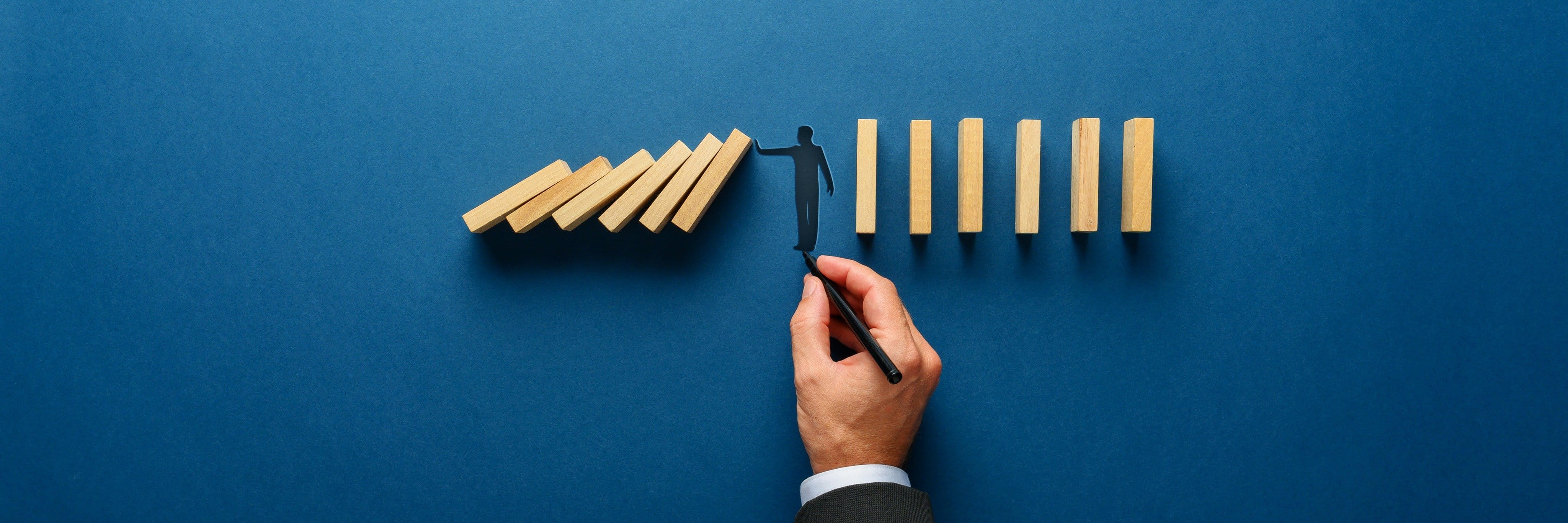
Artificial intelligence and machine learning (AI/ML) is ticking on every business’s radar as their benefits materialize across different industries. While this innovation should be favorably embraced, businesses need to be mindful of the potential risks and unintended consequences of AI/ML adoption, and how to sidestep them.
Opportunities come with risks
In principle, algorithms and domain-specific AI/ML are growing at a rapid scale due to its many advantages. McKinsey Global Institute research suggests that by 2030, AI could deliver an additional global economic output of $13 trillion per year [1]. Its highest utilization is generating logical business-driven predictions, creating a more personalized customer experience, and identifying fraud in real-time.
Although more AI/ML wins are out there for the taking, businesses are seeing its use cases bogged down with a host of unwanted risks. Increasing interconnectivity and convergence comes at a cost as connected systems are always vulnerable to potential attacks. According to the Allianz Risk Barometer 2021, the implications of AI, ML is among the seventh top business risks, ahead of political risk and climate change [2]. Beside that, companies might face new challenges as workloads shift from human to machine. In fact, an Allegion study found that 81% of AI-adjacent industry employees admit training AI/ ML systems with data is harder than expected [3]. These data-related problems could stem from how data is being produced and studied, including bias and compliance issues.
The underlying spectrum of threats
Businesses can have a firmer grip on AI/ML development by pinpointing the following five areas for addressing threats:
- Software accessibility: The main component of AI/ML is software. These easily available, open-source softwares allow any user to see, study, modify and enhance as they fit. As a result of its open nature, injection flaws - which enables an attacker to move harmful code from one program to another - might be the most imminent risk of software vulnerability. Beside that, calls to the operating system, use of third-party programs via shell commands, and SQL injection are all possible dangers.
- Liability: While AI/ML agents could take over many decisions from humans, they are not legally liable for said decisions. Generally speaking, the unit in charge of utilizing these tools is likely to be held liable for AI-driven system defects. Taking the healthcare industry for instance, when health-care AI systems injure patients, whether due to bad manufacturing, design, or failure to notify users about potentially dangerous situations, developers risk facing product liability lawsuits.
- Ethics: Ethical concerns are more and more important once AI/ML pervades society. AI can decrease human subjectivity in predictions and decision-making, but it can also include biases, resulting in erroneous and/or discriminating predictions. Not only could AI replicate human biases, but it also gives them scientific legitimacy. It creates an illusion of objective forecasts and judgments, while the fact is much the opposite.
Mitigating risks through AI/ML governance
By using a structured risk management framework (RMF), business can map out the most critical threats underlying its system, and thereby govern it better. An AI/ML RMF could begin as a continuous cycle with four steps as below:
- Identify: As the AI model learns and evolves, companies will need to conduct periodic reassessments to see if the risk profile of an AI use case has changed. The identification of AI risks should include risks enterprise-wide. Data ethics, privacy rights, and applicable regulatory considerations should be addressed in the auditing process; Along with questions of whether the data input is suitable, or appropriately safeguarded (via access right controls and encryption protocols, for instance).
- Assess: Because AI models evolve over time, companies may discover that the initial definitions and assessment metrics are insufficient to address the model's decision drivers. As a result, the evaluation process will need to be more regular and dynamic, and it will need to be reviewed both "bottom up" (for each particular use case) and "top down" (overall risk appetite). The model's technical characteristics, such as bias and classification error, should be assessed. The same goes for the business (such as the number of policies generated by client segment) and operational parameters (e.g. the speed of policy written from initiation to issue).
- Control: The control procedure should take into account how AI interacts with stakeholders (clients, underwriters) across all touchpoints. Should businesses keep track of the whole customer journey frequently enough, they could discover and, if necessary, correct abnormalities and outliers early on. Firms should also have a well-defined "hand to human" process in place – that is, when the AI solution cannot generate a result within the set risk tolerances, human expertise is required.
- Monitor and report: The relevance of the limits and targets connected with AI solutions (e.g. Key Performance Indicators - KPIs) must be monitored at length. The reports should contain both the model's technical performance, business and operational outcomes. All legal and regulatory developments that necessitate a modification in the model's architecture must be considered, as well as external events that indirectly feed into the data consumed by the model.
Building risk management
Another strategic play worth business pursuing is to minimize AI bias in any ethical dilemmas, which would span over three pillars: teams, AI model and corporate governance.
- For teams: Businesses should enable multidisciplinary teams to collaborate on algorithms and AI systems. Not only that, creating a culture that encourages individuals and teams to prioritize equity at every stage of the algorithm development process is another step to counter organizational bias and subsequently AI stereotypes.
- For AI models: Developers must ascertain that dataset development is collected responsibly, with regular checks and balances in place for both developing new datasets and altering old ones. Furthermore, policies and procedures that allow for responsible algorithm development should be created to better regulate AI models.
- For corporate governance: Corporate governance for responsible AI and end-to-end internal policies should be established to prevent prejudice. At the same time, engaging corporate social responsibility (CSR) that drives responsible / ethical AI, as it should be recognized as a top-management issue for its implementation to be effective.
With these actions, organizations will be better positioned to manage AI risks in a more nimble manner, thereby reaping the full benefits of AI/ML adoption.
Reference:
[3] https://insights.sei.cmu.edu/blog/three-risks-in-building-machine-learning-systems/