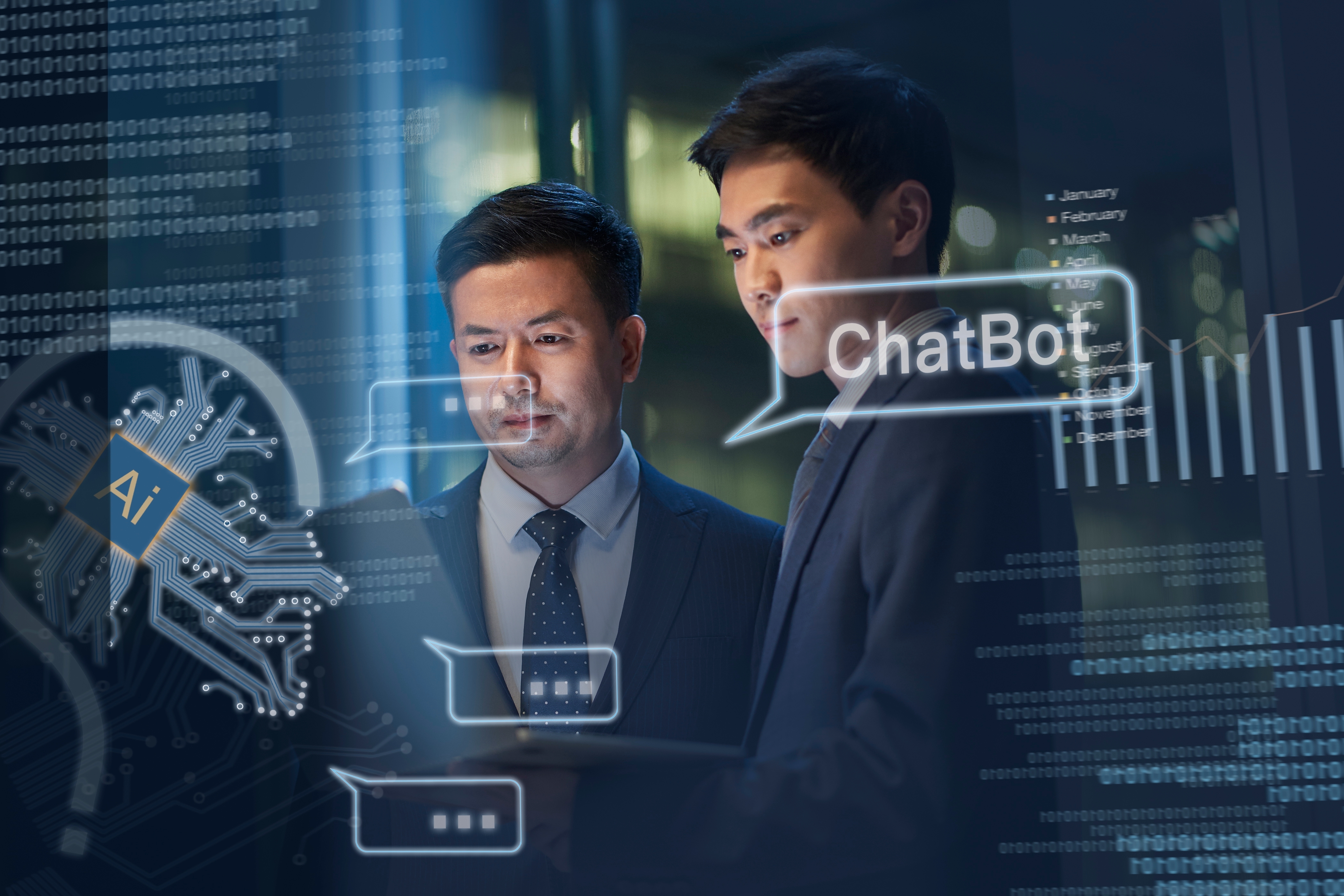
DeepSeek and a turning point in the AI race
Over two years ago, ChatGPT made headlines for being one of the most powerful Gen AI chatbots humans had seen. Today, DeepSeek, a Chinese tech start-up, has stirred up the entire AI world by introducing a similar model with competitive performance but only at a fraction of ChatGPT’s cost. The company claims that its DeepSeek-R1 model is 20 to 50 times cheaper to use than its OpenAI o1 counterpart, depending on the tasks [2]. For training, the company’s own report indicates that its V3 model cost just US$5.6 million to train, which, if proven true, would show impressive cost-effectiveness compared to its competitors’ billions of investment in AI training [3].
The sudden success of DeepSeek surely causes concern for its rivals. In response, OpenAI announced that it would speed up new product releases, with its latest issue being the “Deep Research,” a report generation tool that matches a human analyst’s performance, powered by its most cutting-edge model – o3 [4]. Just a few days prior, OpenAI released the o3-mini, a more cost-efficient version of its powerful o3 model, with matching capabilities to its predecessor in math, coding, and science, but with significantly lower cost and faster response [5]. And OpenAI is not the only one to respond as DeepSeek’s own domestic rivals are also stepping up their AI game. Alibaba, a Chinese tech giant, recently released its newest Qwen 2.5-Max model, claiming to be more powerful than DeepSeek’s V3, OpenAI’s GPT-4o, and Meta’s Llama-3.1-405B. Performance aside, Chinese tech companies are also competing on price, with Alibaba’s cloud unit cutting as much as 97% of prices on a range of models [6]. This situation has sent a clear message: competing with pure power is no longer sufficient.
A heightened focus on open-source AI
One of DeepSeek’s success factors lies in the open-source approach that it follows. An open-source AI system allows everyone to freely access and study how it works, benefit from its functions without limitations, and even modify and build upon the system to serve one’s unique requirements without any restrictions. In contrast, closed AI, or proprietary AI, keeps the source code and algorithms hidden, restricting unauthorized users from modifying the models. With free access, open-source AI benefits from community contributions, helping the AI systems improve continuously, thus lowering the cost of development. In addition, users are able to modify open-source AI systems to serve specific business needs without hefty upfront investment, proving to be an attractive option for those seeking low-cost AI solutions. In the case of DeepSeek, following an open-source approach allows developers to leverage existing open research and open source to build its model, and in turn, enable others to benefit from it. Indeed, it became the top free app in the US within just a few days of release and has witnessed more than 700 derivatives [7].
With DeepSeek’s success, open-source AI has attracted global attention, not just limited to Chinese and US companies. OpenEuroLLM, a new alliance has recently emerged in Europe, aiming to develop high-performance, multimodal, open-source large language models (LLMs) for text, speech, and structured data [8]. The initiative calls for balancing rapid innovation and ethics by pushing AI sovereignty, in which investment in domestic labs and data centers is encouraged [9].
The hidden costs
Open-source AI certainly accelerates innovation with lower cost, yet questions remain on whether it comes at a hidden cost of compromising security. A primary argument has been that the openness of these AI models leaves the system highly at risk of security breaches, as anyone can access and modify the source code. Indeed, researchers have found that open-source AI models are susceptible to several privacy attacks, including model inversion (expose training data by creating output samples), membership inference (expose whether specific data is used for training), and information leakage by memorization (accidental leakage when models memorize and reproduce training data). In addition, the researchers have also found open-source data to be at risk of falling victim to several security attacks, such as data poisoning (manipulating training data to change the system’s behaviors), backdoor attacks (insert hidden functionality in training data and alter the system’s behavior when triggering a specific input), and adversarial examples (craft input data to trick the systems into making wrong predictions) [10].
And one of the most popular open-source AI models, if not the most, DeepSeek, is already being called upon to address security concerns. Italy's data protection authority blocked DeepSeek’s chatbot in January after the company failed to answer the watchdog’s concerns, particularly over the types and sources of data collected, the purposes and legal basis of such data, and whether it is stored in China [11]. Following suit, Australia, Taiwan, and South Korea have also banned state employees from using DeepSeek on government devices. Other countries in Europe, along with the US and India, are also considering similar restrictions [12].
What does it mean for businesses?
The security and privacy concerns do not necessarily mean businesses should turn their back on open-source AI systems. The fact is that even proprietary AI models such as ChatGPT encountered similar concerns and even temporary restrictions from several governments. With immense potential including accelerated innovation and reduced development, it would be detrimental for businesses to overlook open-source AI models. Nevertheless, businesses are advised to cautiously implement risk mitigation strategies, including developing strict internal security policies and ensuring compliance by running the models in a secure and isolated environment [13]. Businesses, especially those that deal with sensitive data including healthcare and financial information, should evaluate AI service providers’ compliance with local and international regulations and standards, such as GDPR and HITRUST. Lastly, considering AI vendors’ transparency and responsible development strategies is equally important to mitigate against privacy, security, and regulatory risks. Collaborating with organizations that prioritize responsible AI, such as FPT, who is part of an active community for responsible AI research & development with Mila, Landing AI and the AI Alliance, is highly encouraged.