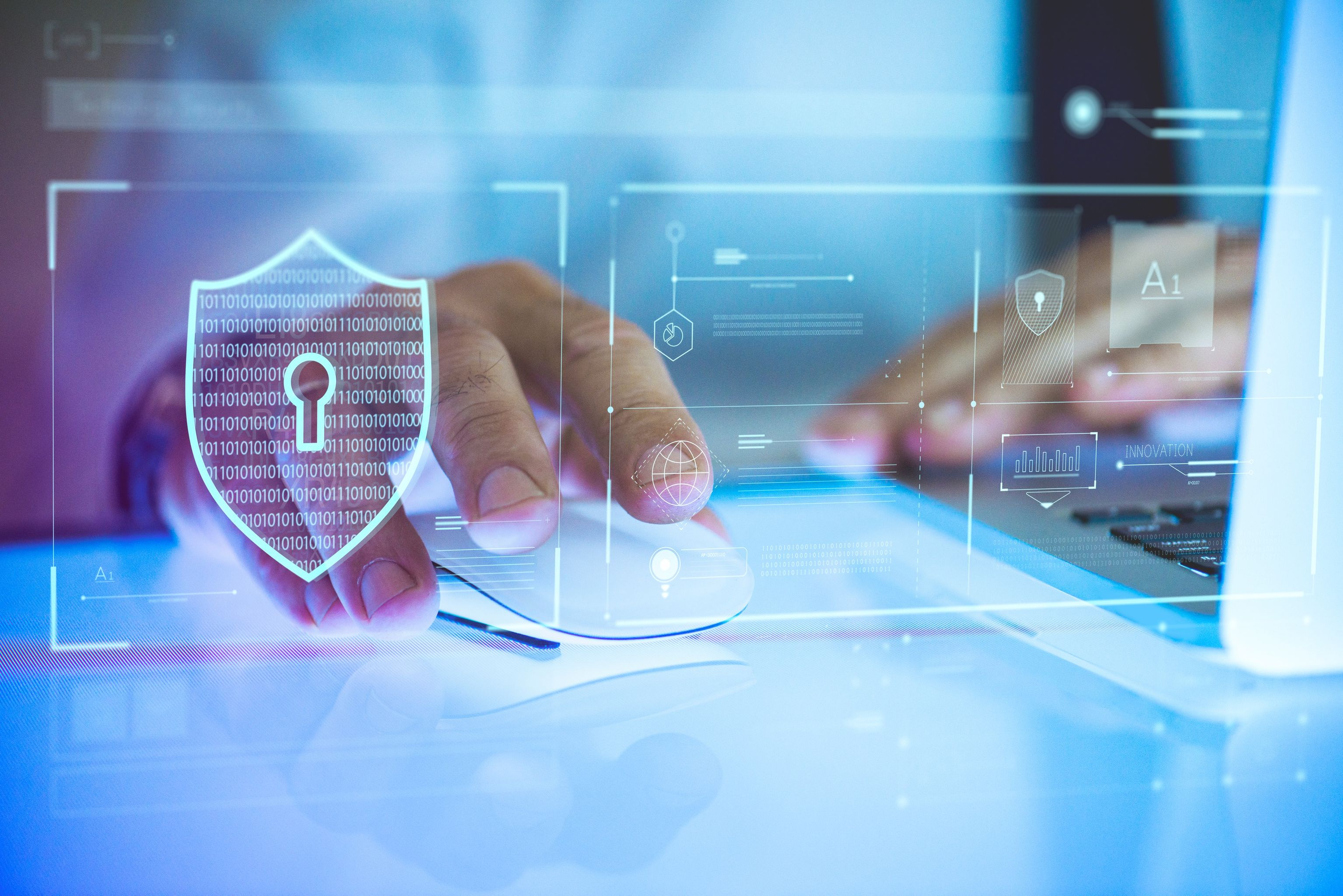
Risk management is one of the most critical procedures that determine every business's competitiveness and innovative stance. With numerous technologies maturing in parallel, the stage is set for a new strand of innovation in risk management: AI and cognitive computing.
The rising popularity of AI and cognitive computing
With its potential contribution of $15.7 trillion to the global economy by 2030, Artificial Intelligence (AI) is hailed at the forefront of technological innovations [1]. Its benefit lies in the cognitive capabilities of any AI model, which includes data mining, machine learning (ML), and natural language processing (NLP). Together, these technologies could train computers to recognize, identify, and assess risks to power business performance.
In risk management, AI/ML has become synonymous with enhancing efficiency and productivity while lowering costs. AI/ML can process and analyze enormous amounts of unstructured data faster while requiring minimal human participation. As a result, The technology produces significant amounts of timely and reliable data that allow institutions to build insightful customer intelligence, ensuring successful strategy implementation at a lesser loss.
NLP is an AI cognitive technology that uses powerful algorithms to analyze unstructured data and generate corresponding insights. NLP technology advances have facilitated a range of activities for enterprise risk management. This includes text extraction and summary, voice surveillance of stakeholders, compliance or fraud monitoring, sentiment analysis in social media, and other communication channels. By incorporating NLP technology into their operations, risk managers can save up to 50% in time spent on information gathering tasks. [2].
Cognitive analytics - the new wave of data analytics
Cognitive Analytics is conceptualized from the combination of Artificial Intelligence (AI) and Data Analytics, which enable systems to process and act on data in a human-like manner. According to a 2019 report by the International Data Group, approximately 90% of data collected is unstructured, and implementing cognitive analytics can put firms ahead of their competitors [3].
Cognitive analytics is transforming industries radically, and its prominent usage has taken off in the banking and finance sectors. With access to new analytic insights, the cognitive bank filters, and digests dynamic internal/external data from the banking ecosystem and beyond. It can capture the "market DNA," including, for example, information about industry trends, financial performance, market risks, and benchmarking analytics. Such proactive data capture and processing help provide compressed cycle times, reduced errors, better compliance enforcement and higher productivity. Already taking strides in CA, 58% more bank leaders (than underperformers) said they expect cognitive computing to transform their business lines and functions significantly [4].
AI in risk management - top use cases
The following are some examples of specific use cases that have benefited from AI integration with risk management solutions:
- Threats management: Large amounts of data from external sources are analyzed using machine learning. Risk managers and security teams can then use the real-time prediction models generated from this data to address IT security issues promptly. These prediction models serve as the cornerstone for early warning systems that assure continuous business operations while boosting data privacy and security.
- Fraud detection: Identifying fraud or theft traditionally necessitated lengthy analysis methods, especially for financial institutions and insurance firms. AI-enabled risk management systems can significantly reduce the workload of fraud detection processes by using ML models trained on social media analysis, text mining, and database searches.
- Data classification: Based on recognized content categories, specified regulations, and trends, AI-dependent cloud engines can identify and tag every data uploaded or generated in a cloud environment and then monitor for access. This makes them the perfect partner for IT managers to detect and quickly correct changing data anomalies, and systematically store data for easier retrieval and speedier regulation compliance.
Emerging challenges of AI application in risk management
Dangers and difficulties always accompany benefits. The same can be said for AI-assisted risk management solutions. When using AI technology, businesses must pay close attention to the risks that come with it, such as data inconsistency and model complexity.
AI applications are programmed to learn from the supplied data, either as a one-off at the time of their design or on a continuous basis, to refine the decisions pattern made over time. This means that the quality of any decision made by an AI solution depends greatly on the quality and quantity of the data used. An absence of large sets of high-quality data is, in general, one of the major obstacles to applying AI solutions. Especially for financial service firms, this is exacerbated by the prevalence of legacy systems and organizational silos, which may prevent the seamless flow of data and/or affect its quality.
AI solutions that learn, evolve, and contain hidden decision processing layers can make auditability and traceability challenges. In addition, the speed at which AI solutions learn and develop can result in errors manifesting rapidly on a large scale.
Looking forward
AI will increasingly become a core component of many FS firms' strategies to deliver better customer service, improve operational efficiency and effectiveness and gain a competitive advantage. It's no surprise then that demand for cognitive computing technologies is skyrocketing. Estimates from Deloitte suggest that the global market revenue for AI-driven cognitive solutions will exceed $60 billion by 2025 [5].
Source:
[1] https://www.pwc.com/gx/en/issues/data-and-analytics/publications/artificial-intelligence-study.html
[3] https://www.itbusinessedge.com/it-management/ai-risk-management/
[4] https://www.ibm.com/thought-leadership/institute-business-value/report/cognitivebank