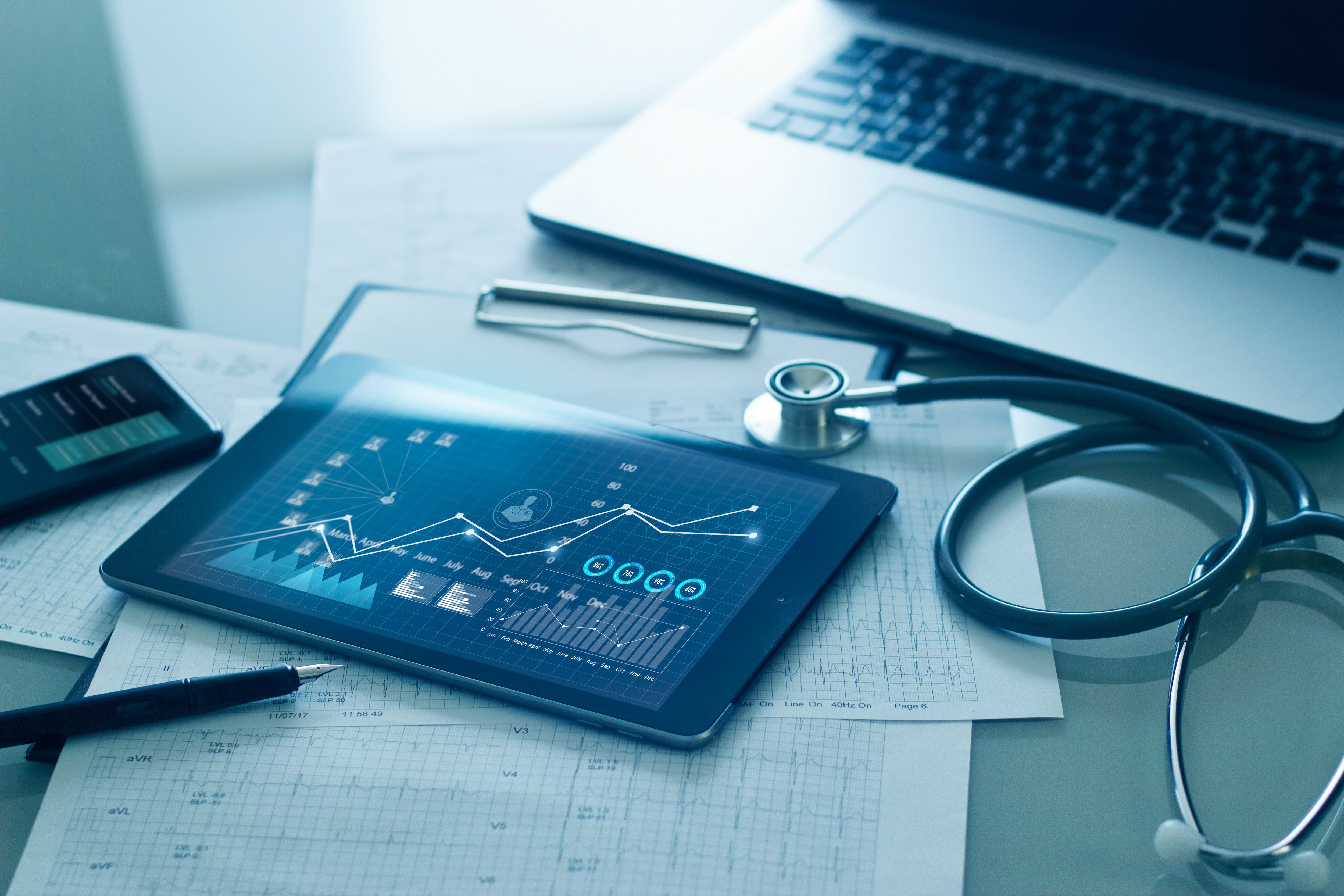
Data in healthcare are long reserved in papers and hard copies, from small reservation tickets to extensive medical records. Since the beginning of digital healthcare in the early 1960s, global healthcare providers have undergone 3 waves of IT evolution. In this modern day and age, these organizations rely on digital records not only to preserve but also to decipher the patterns and trends that contribute to optimized healthcare delivery.
The current state of data analytics in healthcare
a. Market sizeThe current market size of healthcare data analytics records consistent growth despite the COVID-19 pandemic or the economic downturn. In 2023, this industry surfed to 43 Billion Dollars, increasing 14% compared to the previous fiscal year. In the upcoming years, the global market will receive a steady CAGR of 15.9% from 2023 to 2030, resulting in 121 Billion Dollars in 2030. The United States is the leading market for this era, with USD 25 Billion in 2022, making up 68% of the global revenue [1]. In the bargain, despite a humble market share and limited resources, Asia Pacific is the fastest growing market, promising to bypass other counterparts to become the leading region. A large young population with a Hyperconsumerism lifestyle is one crucial factor in explaining this phenomenon. With Hyperconsumerism, younger generations tend to buy fitness wearable devices to not only track their daily health but also express their modern urban lifestyle. Countries like China and India, with more than 2 Billion population combined, are also promising a potential elderly care market in upcoming years. Moreover, many experts acknowledged the rapid recruitment of patients and quality personnel, lower costs, and excellent infrastructure in Asia-Pacific are fundamental for this robust growth.
b. ChallengesLeverage Data Value
In this modern era, the value of data has become increasingly important. One significant potential lies in leveraging data usage to optimize revenue streams. The global healthcare data market was estimated to be worth $0.4 billion in 2023 and is projected to reach $0.9 billion by 2028, growing at a CAGR of 18.5% [2]. In the healthcare sector, the data revolution has led to the emergence of new vendors facilitating data exchange between institutions. These vendors often employ federated learning or synthetic data, transforming how data sharing is managed globally. These techniques require a massive data lakehouse from real-life activities to learn from without exposing sensitive information. As such, healthcare providers can leverage their preexisting data reservoirs and further worldwide technology innovations. To expand business based on existing data, healthcare providers must ensure that their data reservoir is organized, clean, and coherent. Unfortunately, many companies fail to realize the full potential of their existing data, resulting in missed opportunities for direct revenue generation. However, adopting this approach comes with challenges, such as privacy and regulatory concerns.
Talents Scarcity
One of the primary challenges in healthcare data analytics is the need for more talent and the need for proper governance. Talent plays a crucial role in driving innovation and ensuring the success of analytics initiatives in healthcare organizations. However, the healthcare sector lags behind other industries in terms of attracting and retaining skilled professionals with expertise in data analytics and AI development. To thrive in this rapidly evolving field, healthcare organizations must prioritize talent acquisition and establish partnerships with reliable technology providers.
Manage Unstructured Data
Cleaning, analyzing, and managing unstructured data present significant challenges in healthcare data analytics. The healthcare sector generates enormous amounts of data, including structured and unstructured formats, from medical centers, wearable devices, social media, and advanced imaging devices. However, approximately 80% of medical data remains unstructured and unused, making it challenging to incorporate into Electronic Medical Records (EMRs) or hospital information systems [3]. This unmanaged unstructured data is often ignored or abandoned in medical centers, limiting its potential for analysis.
Typical unstructured data in hospitals are (1) medical video data created from new medical imaging devices, (2) biosignal data displayed on the monitor/wearable device's screen, and (3) audio data created by patients' daily interactions and medical staff. Businesses must establish data collection, anonymization, and quality assurance processes to enhance these disorganized medical data use. And metadata for each type of medical data need to be defined, standardized, extracted, and visualized automatically. Then open platform for integrating and utilizing such clinical data should be developed while reflecting these concepts.
Trending New Applications of Healthcare Data Analytics
Drug Discovery
A medicine development process, in general, can cost businesses up to $2.6 Billion and 12 years of intensive research. While the average time it takes to bring a drug through clinical trials is exhausting, the success rate has been meager to just 12 percent in recent decades [4]. In preclinical drug discovery, researchers identify potential targets for drug development. This stage entails extensive trial and error as researchers test numerous compounds to find those with therapeutic potential. Data analysis can optimize these perplexing processes by evaluating large amounts of biological and genetic data to identify potential drug targets and optimize existing ones. Thus, data analytics will increase the drug delivery process rate in medical science, helping to gain faster approval, especially when pairing up with the implementation of AI for better quality control and coherent management.
According to McKinsey, approximately 270 companies utilize AI in data analytics for drug discovery purposes, and Aramis Biosciences is one prominent example [5]. This biopharmaceutical company, established in 2018 as a spin-off from Harward Medical School, specializes in treating dry eye disease, which affects around 344 million people worldwide. With the aid of the Exscalate supercomputing drug discovery platform, Aramis can detect lead candidate molecules for treatment. The Exscalate system consists of three main components: (1) a ligand library containing over 2 trillion organic molecules, utilizing AI to analyze the correlation between molecular structure and activities; (2) a database of therapeutic targets; and (3) a ligand generator for conducting simulations on supercomputer clusters. By doing so, Aramis has completed the project within 14 months compared to traditional methods that typically take years. Aramis, currently, has finished enrolling participants for Phase II clinical trials of their dry eye syndrome treatment agent.
Enhancing Patients Experience
Data analytics has found interesting applications in treating home-based patients, particularly in managing post-surgery complications and recurring pain patients often experience after leaving the hospital. By utilizing data analytics in remote in-home monitoring, doctors can quickly diagnose symptoms through extensive data analysis, reducing the reliance on expensive physical resources. Through monitoring devices such as Apnea monitors, Fetal monitors, or Breathing apparatus, healthcare providers can remotely analyze caloric burn, ECG (Electrocardiogram), respiratory rate, or sleep patterns data generated via such devices for better accuracy and convenience. By analyzing continuous health data, doctors can detect early signs of illnesses and provide timely interventions, potentially preventing the progression of diseases and reducing the need for hospitalization. Moreover, aggregated data from mass patients can be analyzed to identify trends in specific populations and inform public health initiatives and preventive strategies.
In the bargain, doctors can now perform faster diagnoses and prescriptions or even conduct consultations via telemedicine thanks to constant analysis of daily patient activities. Moreover, for patients in need of acute medicine or general medicine (which could take up 23% and 19% of readmissions, respectively [6]), data analytics can accelerate the delivery process by examining previous patients with similar symptoms or conditions to provide faster results. Hospitals are also expected to leverage electronic medical record (EMR) data to predict readmission requirements within a shorter timeframe in upcoming years.
Conclusion
Data analytics has emerged as a game-changer in the healthcare industry, enabling organizations to make informed decisions, improve patient care, and drive innovation. By overcoming concurrent challenges and leveraging the emerging applications of data analytics in drug discovery, post-care monitoring, and federated learning, healthcare organizations can unlock new opportunities and drive positive change in the industry.