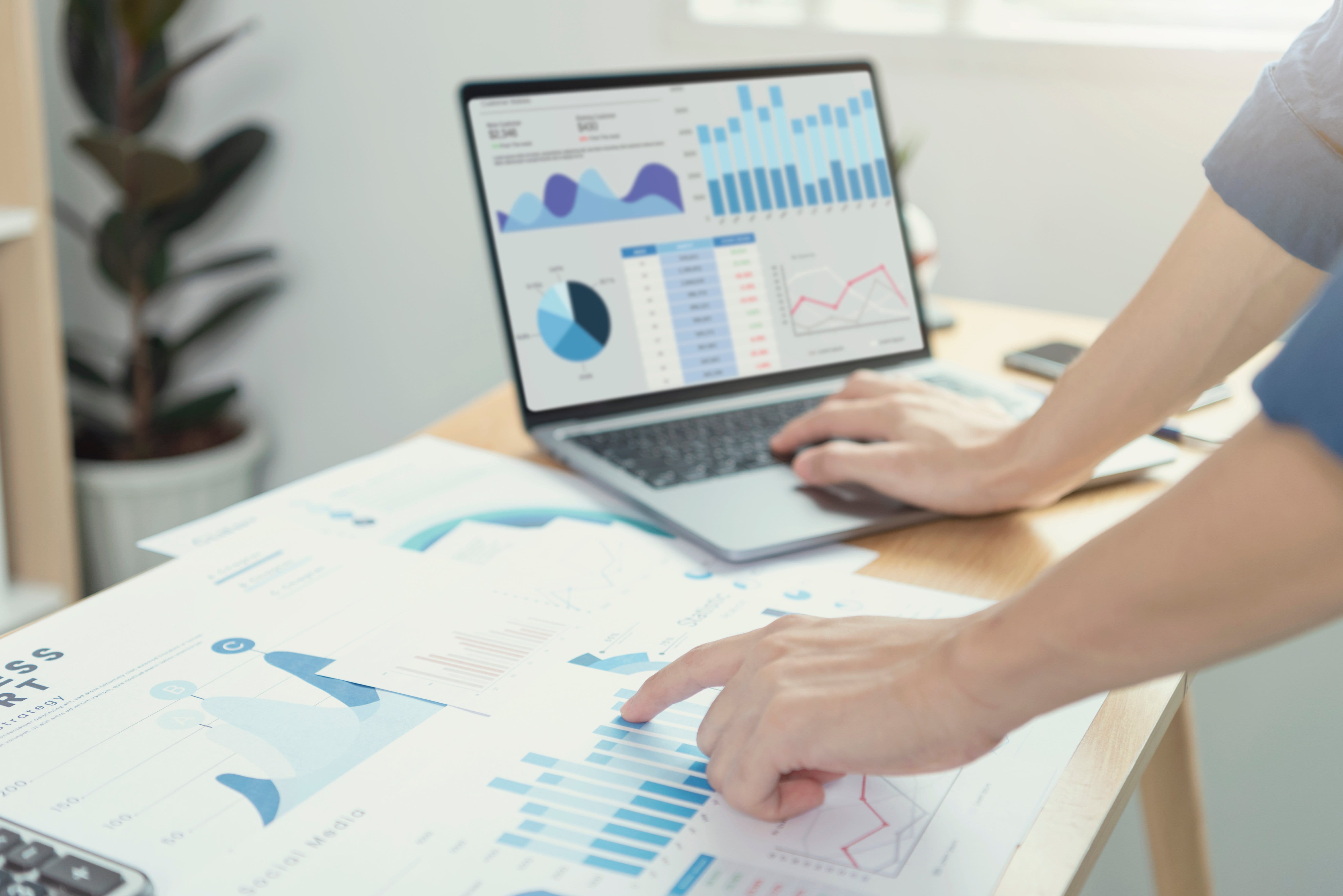
Data is the bleeding edge of survival for businesses. As technology companies find themselves at the crossroads of changing market dynamics, observing and adapting to emerging data analytics trends is imperative. These trends include:
- Data mesh;
- Data-as-a-Service (DaaS);
- Data democratization, and
- Synthetic data.
1. From data mess to Data Mesh
Data mesh is a new approach to managing and utilizing data within organizations. Unlike traditional centralized data models, data mesh decentralizes data ownership and distribution. The main idea is to enable different teams to take ownership of their data, making data-driven decisions independently. As only 32% realize tangible value from data [1], data mesh is a solution for businesses to manage their data in a scalable manner.
Adopting a data mesh approach offers a range of significant advantages for businesses:
- Adaptability: As data mesh employs a decentralized structure, it can facilitate robust data management practices that seamlessly accommodate growing data demands.
- Avoid bottlenecks: Data management teams might be overwhelmed by data requests from across the organization. By distributing data ownership and management to domain-focused teams, data requests are directed to the pertinent teams, reducing wait times.
- Customization: This decentralized structure encourages customization, allowing each team to employ tools and technologies tailored precisely to their requirements. This individualized approach promotes data analysis and processing efficiency, where data-driven insights can be quickly translated into actionable strategies.
2. Data-as-a-Service (DaaS) is here to stay
As more and more organizations turn to the cloud to modernize their infrastructure and workloads, data as a service, or DaaS, is becoming popular. DaaS is a data management strategy that uses the cloud to deliver data storage, integration, processing, and analytics services via a network connection.
DaaS offers seamless implementation, requiring just minutes to integrate into existing systems. DaaS streamlines maintenance through automation, eliminating the need for users to invest time in routine tasks like backup storage, as the DaaS provider ensures constant updates. This leads to reduced staffing needs, as providers handle many tasks. Moreover, DaaS proves cost-effective by minimizing expenses associated with storing and upkeeping intricate systems and databases. It also reduces IT costs by transferring infrastructure maintenance to service providers, with pricing mainly determined by the data storage capacity.
The banking and financial industry faces the critical task of accessing, analyzing, and acting on the data generated in real time. Interestingly, it has been observed that only big national and regional banks prioritize the need for data and analytics. Also, the presence of firms involved in financial analysis or stock markets is expected to benefit from DaaS primarily.
In the future, DaaS is here to stay. This significant rise in the total value of investments into fintech companies worldwide will offer the market a wide range of lucrative growth opportunities. With a predicted market size of USD 56.85 billion before 2027 [2], driving the market's growth considerably throughout the forecasted period.
3. Improving transparency with Data Democratization
In the past, information was closely guarded, resulting in the emergence of data democratization. This data approach empowers end users to access the required insights without relying on IT to grant access. As a result, data democratization creates a culture of transparency within the organization, where information is shared freely, and everyone has a stake in the company's success. This not only improves productivity but also contributes to business efficiency. According to a Harvard Business Review survey, 97% of business leaders considered democratizing data important to the success of their business [3].
Data democratization will witness further growth in the healthcare industry and customer-facing departments. Medical data exists across disparate healthcare systems, and most patients cannot view their comprehensive data records. Even though health records can be shared, this is often a labour-intensive process for patients and medical staff, prone to errors and oversight. This movement towards data democratization aligns with the fact that over 77% of businesses have reported an upsurge in their accessibility to valuable data [4]. Consequently, data democratization can change how corporations manage, share, and utilize data to enhance transparency.
Technological advancements continue to impact how data is collected, analyzed, and used. As a result, companies will need to adapt their data practices to meet these expectations. The emergence of new data privacy regulations, such as the General Data Protection Regulation (GDPR) and the California Consumer Privacy Act (CCPA), reflect this trend and will likely lead to further changes in data collection and management practices.
4. Utilizing Synthetic Data with machine learning algorithms
Synthetic data, which is artificially generated rather than derived from real-world events, is a valuable resource for various applications. It is algorithmically produced and is an alternative to actual data in test scenarios. Its primary functions include validating mathematical models and training ML algorithms. Leveraging a database to create a machine learning model can subsequently generate a secondary dataset from this model.
For organizations that prioritize data privacy, synthetic data can be an advantage. Unlike attempts to anonymize data, which can often be ineffective, synthetic data eliminates this concern by never being sourced from real data in the first place. As the use of synthetic data grows, Gartner forecasted that a substantial 60% of the data employed by AI and analytics solutions will consist of synthetic data [5].
Specifically, synthetic data is highly critical in the medical field. UC Davis Health in Sacramento, California, secured a USD 1.2 million grant to pioneer innovative methods for generating synthetic data, helping to project disease incidences [6]. In another instance, a German health insurance group named Provinzial has harnessed synthetic data for predictive analytics. Their application involves constructing an analytics recommendation engine capable of foreseeing the services and products their clientele might require. By adopting synthetic data, the company saved 3 months by circumventing privacy evaluations and reducing their time-to-data by 4 weeks [7].
Towards a data-driven business
Data analytics will continue to play a vital role in the future for corporations, regardless of business size or industry. With various data models available, companies will continue to rely on data analytics for security and strategic decision-making.