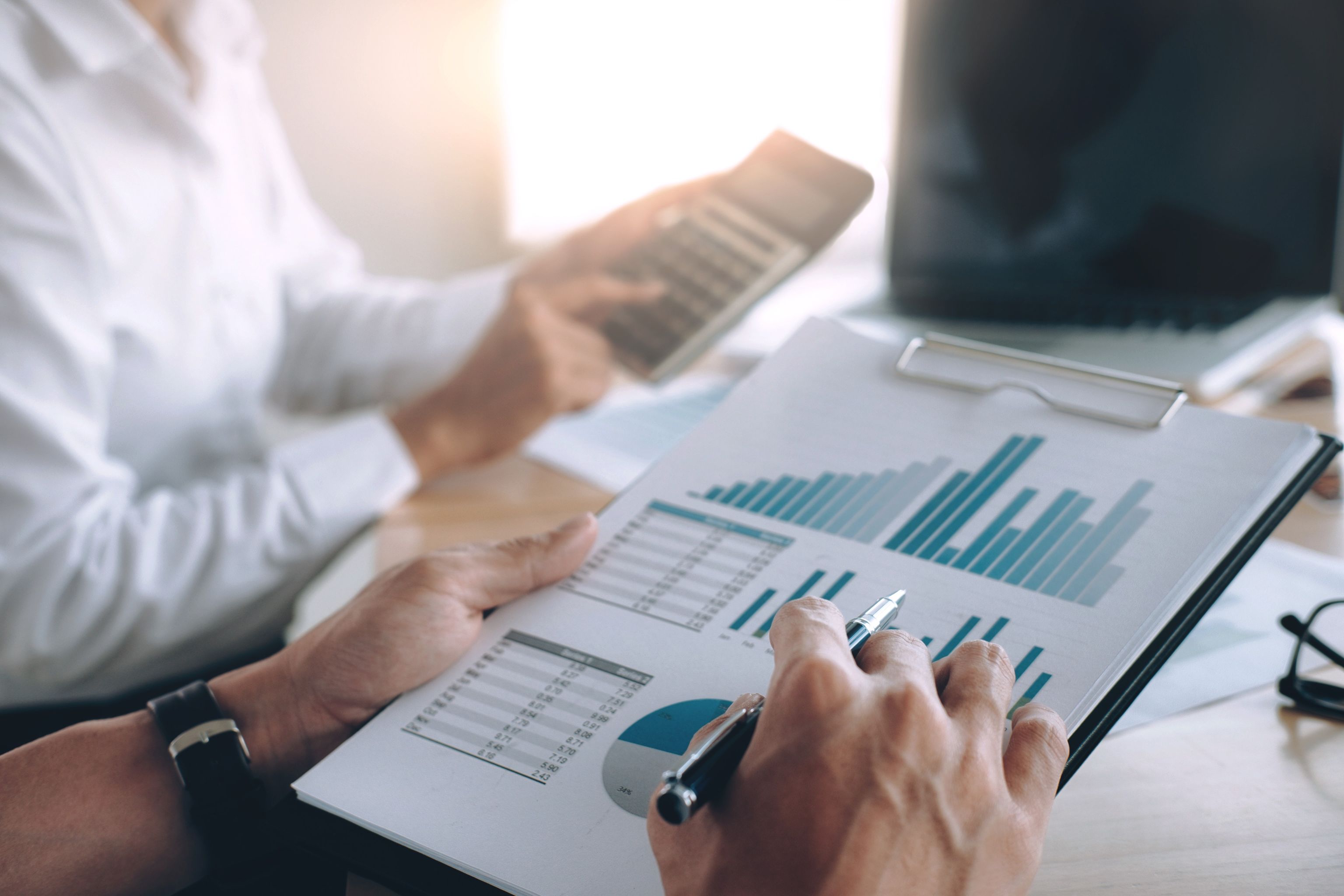
2020 saw banks struggle with the impacts of the pandemic and lose focus on the technology race. The global banking and security sector’s spending on IT in 2020 was relatively unchanged compared to 2019 (US$539 billion and US$542 billion, respectively). Nevertheless, as the outbreak is somewhat controlled, the race is slowly back on track with global IT spending by the sector increasing 5.7% in 2021 [1]. What’s more, banks are expected to further accelerate their digital transformation in 2022 by having a combined IT spending to grow double-digit, according to Forrester [2].
Here are technologies that could speed up banks’ recovery:
Robotic Process Automation (RPA)
Cost-cutting becomes a go-to post-pandemic strategy for banks. 61% of surveyed companies set it as of higher priority compared to pre-COVID period [3]. With the potential to save human effort by 70% and cut processing time by 90%, it is no doubt that RPA is critical for banks to achieve cost initiatives. According to a survey conducted by KPMG, a whopping 90% of participating banks see RPA as key to cost-cutting [3]. Indeed, with its ability to automate manual, repetitive processes, the application of RPA is endless, from updating customers’ information, processing CITAD to tracking and suspending suspicious transactions.
Want to further explore RPA in banking? Check out this infographic here.
But the true potential is only unleashed with hyper-automation. To put simply, hyper-automation is the act of combining RPA with other next-gen technology and allows banks to automate more complex tasks beyond repetitive, low-value works. Take the labor-intensive, time-consuming regulatory reporting as an example. This process requires a concerted effort from different departments to consolidate an enormous load of data from multiple sources, then translate and report in various formats. Using RPA in conjunction with AI can prove helpful in this case, from automation of data extraction, cleansing and validation to report review and analysis with Natural Language Processing (a subfield of AI). These areas maybe highlighted by banks due to its higher ROI, but experts expect the process of regulatory reporting can be automated end-to-end with hyper-automation [4].
Artificial Intelligence
Artificial Intelligence could be at the forefront of banking innovations, from transforming customer experience, aiding decision-making, improving cost management to generating new revenue streams. In fact, McKinsey estimates that the global banking sector can benefit from AI application with an additional US$1 trillion worth of value annually. It is undeniable that the customer experience has changed at an unprecedented rate, and was further fueled by the pandemic. The consequent lockdowns have driven consumers online – on a global scale, 15 – 45% of customers plan to reduce branch visits, even when the outbreak is over [5]. This has raised the customers’ digital expectations, and the unwanted competition from tech giants even set the bar higher. It has come to the point where customers expect personalized suggestions for needs they are not yet aware of. Here is where AI can benefit the front offices, offerings feature such as analyzing transaction data and personalizing suggestions, allowing biometric authentication and frictionless payment, and providing investment recommendations backed by analysis of the customers.
Generating revenue by gaining insights and uncovering new product offerings is another area AI can tap into. ’ESG financial product’ may be the newest buzzword in the BFSI sector, where investors value environmental, social and governance criteria, and investment products are designed with the philosophy in mind. ESG products have shown increasing popularity, with US investors pouring US$20.6 billion in sustainable funds in 2019, quadrupling that of 2018 [6]. With the growing environmental concerns and ambitious pledges made by world leaders in the recent COP26, it is no doubt that demand for ESG products would increase. And financial institutions will need to jump on board to stay competitive. Nevertheless, offering such a product is no easy task – measuring a company’s environmental impacts, especially indirect ones, is quite a challenge. Even when such data is widely available, analyzing them is simply beyond human capabilities - because by 2025, there could be 160 zettabytes of data globally with 80% of which remains unstructured [7]. Here, AI is essential to process this crazy large amount of data, analyzing its patterns and make predictions of a company’s indirect impacts, which is impossible to directly quantify.
Working in harmony
In spite of its advances, hyper-automation is not a one-size-fits-all solution. Solely implementing RPA or AI without a comprehensive strategy and a supporting infrastructure would be a wasted investment. Technology shines best with the right business model – one that is scalable, agile and flexible – which often are traits that are not inherent to the legacy systems. New opportunities discovered by AI would have been pointless if banks take years to execute. Automation would not go beyond low value-added works. And data analytics would be useless if data takes weeks to collect. Building a strong core system is, therefore, of utmost importance. And pulling effort from different technologies (such as cloud), as well as building the right mindset (such as API-first) - is a good point to start.