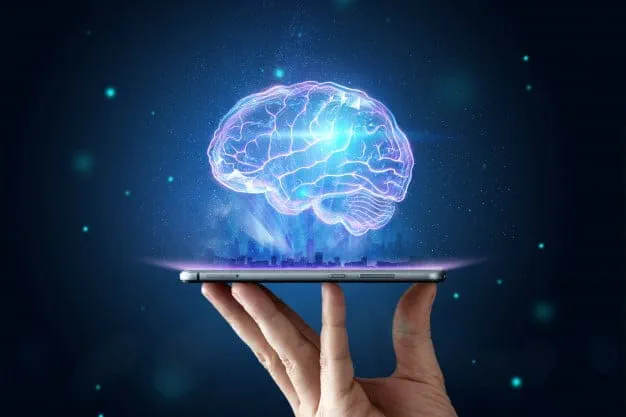
Talents are the power which fuels our organizations. In current highly competitive market, how can we attract, nurture and retain this most valuable asset? The scarcity of proficient resources and the growing expectations of today employee requires an extraordinary approach to Human Resource (HR) management. While specific processes are the responsibilities of HR department, they involves managers in every level in the company. It is thus necessary to provide HR insights from the lowest hierarchical level.
Heavy investments were put into information systems which manage recruitment, performance and employees' development in order to maximize their capabilities. The problem is that they are designed as a one-size-fit-all and cannot generate insights out of multiple data sources.
While the previous article analysed the help of Artificial Intelligence in reading and evaluating candidates, this writing will elaborate the effectiveness of AI in prevent talents from the risk of attrition. The advances of data analytics and machine learning allow us to study data recorded by these tool to generate valuable HR reports. Taking one step further by combining these reports with analysis of HR metrics, we are able to deliver digital transformation for HR management.
A right analytical approach and data utilization may benefit organizations in many aspects including, but not limited to, efficient recruiting, optimized workforce planning, and minimized retention.
1. THREE KEY ELEMENTS OF HR ANALYTICS
Some biggest obstacles lie in the process of extracting real value from an abundance of data. They are inefficient use of data, asking the wrong question, and shortage of analytical ability and domain expertise.
Quality data
The first step of any data science project is data gathering. Thanks to HR databases and employee information systems, we are able to collect and inspect various type of HR data:
The keys to successful analysis is to integrate data from various sources. It is also critical to understand how the data were collected and what they are about. Such processes are difficult and time-consuming, but play a crucial part in producing precise analysis.
There are 3 criteria that need to be satisfied before putting data into effective use:
- Completeness: No missing value in any required field
- Consistence: Data of same format and no conflict between different sources
- Validity: Encrypted data which conforms to a schema and standard with high creditability
Right questions for HR analytics
Before launching any analytical process, the organization should always define the right goal, thus choosing an appropriate approach. Currently, every company are facing a shortage of qualified workforce, while their well-trained seniors are in danger of attrition. How to unleash the potential in new hires, equip them with business know-how and expertise, relocation and promotion plan to save talents, there are questions and associated indicators that need to be clarified to prevent wasting of time and resources.
Using appropriate HR expertise, we are able to provide some practical recommendations:
- Is this employee likely to resign in the next two months
- Which employees should be relocated?
- Negative employees that need special attention and management
- Employees that should be promoted
- Employees that should be given more challenging tasks
- What are the traits of the most productive people
Capable analysts
A typical data science project team often consists of a project leader who works alongside with one or several business analysts, domain experts, data engineers, data analysts, and data scientists. The cross-functional structured allows individual productivity and efficient collaboration. For this highly domain-specific project, people with both analytics capabilities and HR understanding is of critical necessary. Their wide-ranging experience allow them to define a practical and meaningful target for the project, as well as speedup the data mining and feature engineering processes.
Read more: Human Resources Analytics For Saving The Value Of Talents
2. ANALYTICAL APPROACH
Data miningThe keys to successful analysis is to integrate data from various sources. It is also critical to understand how the data were collected and what they are about. Such processes are difficult and time-consuming, but play a crucial part in producing precise analysis.
In this section, FPT Analytics Team brings strong and demonstrated methodology defining how to manipulate data correctly, uncover hidden data patterns to predict present employees in the risk of attrition.
Modelling techniques
The outcome of this phase is not only a predicting model with almost 85% accuracy, but also showing the way to solve this turnover problem by applying data insights.
The problem is converted to a classification problem in which the system will classify an employee into the QUIT or NOT QUIT class at time based on the information about his/her work profile. We can also add working time information to training and testing data to turn this into a time-based classification problem.
In the training process, the data will first be processed, extracted features and then fed into a supervised learning model. The system uses data engineering and feature extraction methods along with classification engines such as Decision Tree, Random Forest, and Support Vector Machine. The output of this model is the confidence score for each prediction it made, or in other words, the probability that an employee is going to resign in the next 3 months, and the important features that affect the decision-making process the most.
3. EXTENSIONS – TO GET EVEN BETTER RESULT
Social listening analysis
In general, Social Listening is not only associated to social media and workplace network, but also describes a way how to explore various type of interaction. Social Listening help us to get more data insights and measure employees performance from useful information like financial stress or dissatisfaction with job parameters. Based on employees activities and reaction with different types of content such as job opportunities, job reallocation, knowledge sharing, etc. from various networks: Facebook, Twitter, LinkedIn, etc., we can come up with many more interesting report and prediction.
Interested in more technology articles? Click here to learn more!
Reference
[1] |
"How to improve brand health with social listening," 14 04 2015. [Online]. Available: https://www.infomart.com/how-to-improve-brand-health-with-social-listening/. [Accessed 20 08 2019]. |