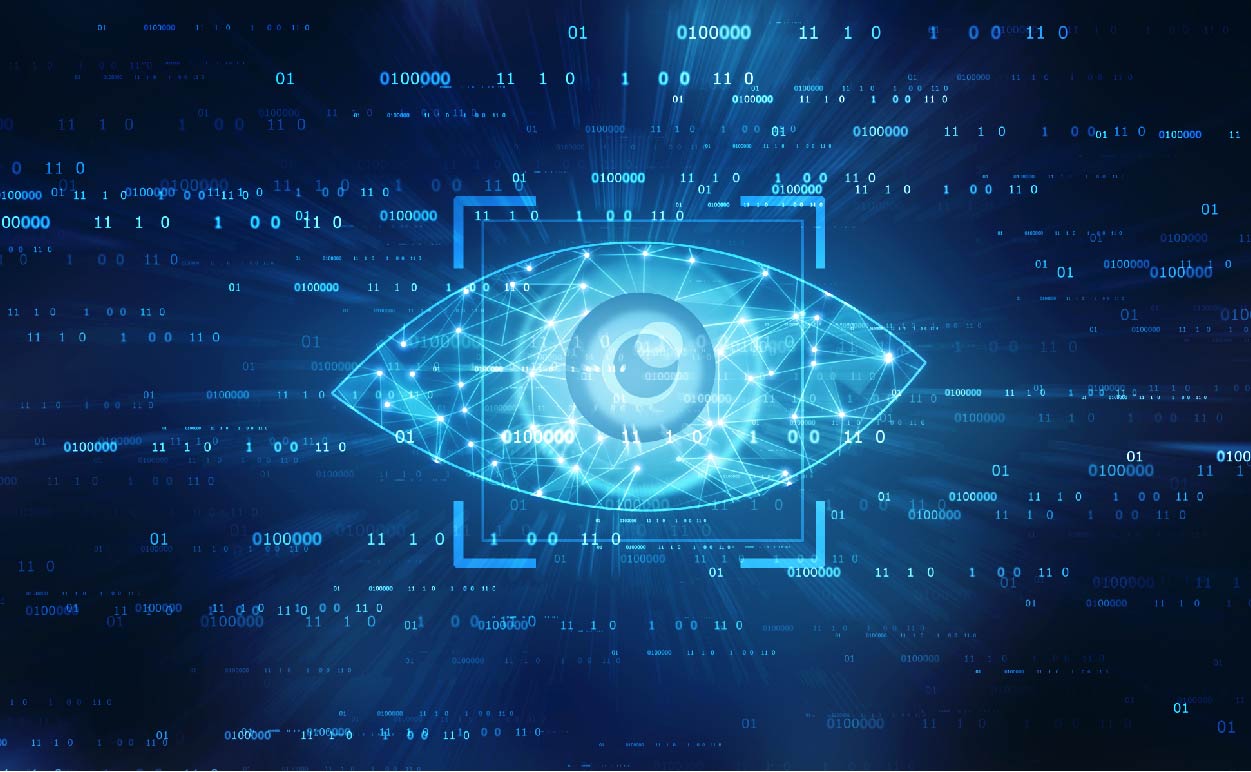
Sports could be a domain where computer vision could make useful decisions.
Computer vision is a subfield of artificial intelligence that uses deep learning and machine learning to interpret the image and video contents. While healthcare, transportation, retail are usually hailed as industries significantly benefiting from computer vision; in reality, sports could also be a domain where the technology could make useful decisions. According to Statista, the sector's computer vision market revenue reached approximately US$1.5 billion dollars in 2019.
The reason is quite straightforward. The sports domain mostly entails fast and accurate motion, which makes it difficult for audiences to follow, and coaches to track and analyze. Monitoring sports movements using sensors and wearables, therefore appears to be impossible. Computer vision, on the other hand, provides a rich set of opportunities for the fulfilment of these tasks.
Ball detection
The first area of sports analytics, where computer vision holds promise is ball tracking. The capability to track a ball in low-latency real-time is crucial for both the broadcast station and umpire. In ball detection systems inspect every camera image available to detect every possible object resembling the typical attributes of a ball (e.g., elliptical shapes in an estimated size range). When the system recognizes such objects, it will build a 3D trajectory of the ball by connecting multiple frames where the ball was spotted to demonstrate its path across several camera angles. The results would help the referee, for instance, to decide if the ball has landed in or out of bounds during the games. Soon, the increasing adoption of computer vision in ball detection might eliminate the need for line umpires.
Ball detection is a computer vision application that is particularly beneficial in sports like tennis, where the ball is visible in most frames. Indeed, Wimbledon is one of the major tournaments that has adopted computer vision for ball detection. However, in team sports, ball tracking using computer vision would be more challenging since multiple players are likely to occlude the ball. This calls for more efforts in advancing technology.
Player detection
Player detection involves the tracking of all players’ positions at a given moment of time. In sports, tracking is particularly challenging since it involves frequent occlusions and abrupt movements; and faces and jersey numbers are only visible in limited cases. Additionally, it is relatively impractical to use facial recognition to track players since image resolution is often too low, even for humans to detect players. This calls for extra efforts in developing new tracking methods.
Computer vision has the potential to improve player tracking. The results gained from a computer vision-based system could be augmented by adopting machine learning. Once key contents in a video or image frame are spotted, semantic information would be generated to establish a context on the actions that players are performing like defend, run, etc.
Motion analysis
Advanced training might be associated with the utilization of motion analysis systems with a wide array of calibrated cameras and markers placed on players. Nonetheless, these systems appear to be inappropriate to apply during actual games. On the other hand, computer vision, which often uses a single camera or a limited number of cameras, is still able to produce illuminating insights into the motions of players at key moments. These insights would help coaches, and industry professionals assess the performance of individual players and the entire team during official games and training sessions. Additionally, the system would provide teams with information regarding their competitors’ tactics.
According to Analytics Insight, the market share of computer vision is projected to reach $16,7944 million by 2025. Given such dynamic growth, the use of computer vision would continue to be a part of the technological evolution of the sports domain.
Interested in more technology articles? Click here to learn more!
References
Lu et al. (2013). Learning to Track and Identify Players from Broadcast Sports Videos.
LinkedIn (2019). Sports Video Analysis using Machine Learning.
Thomas et al. (2017). Computer vision for sports: Current applications and research topics.
Sports Performance Analytics (2020). Computer Vision in Sports.
PwC (2019). Artificial Intelligence Application to the Sports Industry.